Quantum for Finance - An Interview with Carmen Recio.
Dan: Hello and welcome
to the quantum divide.
This is the podcast that talks
about the literal divide between
classical IT and quantum technology,
and the fact that these two domains
need to become closer together.
Quantum networking actually is
more futuristic than perhaps
the computing element of it.
But we're going to try
and focus on that domain.
But we're bound to experience many
different tangents both in podcast
topics and conversation as we go on.
Enjoy.
Hello and welcome back to the
quantum divide in this episode.
I'm pretty excited because we're taking
a step away into some of the applications
specifically one of the applications.
with quantum computing.
I've got here with me, Carmen Recio from
Spain who works for Moody's analytics.
I'm very excited to dive into some
of the aspects of the way quantum
computing is relevant in the financial
sector and risk management and so on.
So let's get started straight away.
I think Carmen.
Welcome to the podcast.
Carmen: Thank you so much.
Very happy to be here.
Dan: Great.
Let's start with an intro.
This is a standard podcast format, right?
If you could give us a bit of a view
on your path into quantum perhaps
through your, education and so on.
And then how you ended up at Moody's.
Great to hear the story.
Thanks.
Carmen: Okay.
Yeah.
Thank you so much for the question.
So actually when I was studying, there was
no formal education in quantum computing.
So my path is that of many
of the people who are working
in quantum computing today.
Actually I studied mathematics in
university and then after, right
after university, I joined IBM, but
I didn't join the quantum department,
I joined the AI hardware department.
And I was working as a data scientist for
some years, but then one day I went to
a lecture, and I discovered that quantum
was something that IBM was doing too.
And I didn't even know that existed, that
a kind of computing that was based in
quantum mechanics, but it got me excited.
And so I asked if I could
do something about it.
And at the time, the only thing you
could do working in IBM from Spain was
becoming part of team that was like a
team of evangelists, but it still was
very cool because you got trained by the
researchers working in the technology.
So that's how I first got into quantum
and I loved it so much that after a couple
of years being an evangelist, I decided
I wanted that to be my full time job.
And I had also been involved a lot in
community activities, not just with
quantum computing, but also with AI.
But thanks to my engagement with
technical communities, where we did
meetups for external people, student
communities that were interested in
learning about new technologies, I got
to meet the team inside IBM Quantum
that was in charge of outreach,
education, and community building.
And we organized the first
hackathon on Qiskit hackathon.
Qiskit is the framework of IBM.
We organized the first hackathon
that had ever happened in
Europe and it was in Madrid.
It was not only because of me, but
because of many of the people working
in Qiskit are actually Spanish too.
That's a curiosity.
So after meeting that team I told
them that I will eventually like
to work full time in Quantum.
And then at some point I was lucky
enough to have the opportunity
to move to Zurich and join the
research lab , that IBM had here.
And I spent there a couple of years
working in outreach, community
education, but also part time with
a group working in applications,
concretely Stefan Werner's group.
That was working in, more specifically at
the time the team was divided like in two
big areas, and Stefan's part of the work,
of the group was working on optimization,
machine learning, and financial
applications of quantum computing, and
this is how I got into quantum finance.
And after some time, I changed roles
and I and also companies and I joined
Sergio Gago's team in Moody's to take
on a new challenge and change from
a company that was on the offering
side of quantum to a company that was
in the application side of quantum.
And I was very excited to take
on that challenge in order to
see another dimension of quantum
computing, which in this case are
applications in the financial sector.
Dan: Fantastic.
Data science so it must be some
interesting way of applying that
to or applying quantum to solving
problems in data science, right?
Or at least tackling, tackling problems.
Yeah, why don't you give us an idea
on some of the challenges that you
see , if there's some kind of general
problem sets that you get with financial
organizations or financial data that
you think quantum would be relevant for
Carmen: Actually, there are
many good studies on that topic.
Concretely there is a survey of quantum
computing use cases in finance that
was published early in 2022, and there,
Marco Pistoia and other researchers
were the first to classify what are the
three big areas that we find the problem
categories Finance where we potentially
will have some advantage from quantum.
And these three areas are a
stochastic modeling in which
you find classical problems as
derivative pricing or risk analysis.
Then you have a second
area, which is optimization.
So anything that has to do with
optimization that Is a category that
is common to many fields, but also to
the field of finance, like a very well
known example is portfolio optimization
and the last one would be machine
learning with all the things that you
may want to do anomaly detection for
financial crime and what other examples
like risk clustering, there are many,
but . Right now, what we are working on
and what we want to guide our clients
or work with our, together with our
clients in is basically three key areas.
So the first one would be post quantum
cryptography, because quantum computing,
as most of the people know probably also
in the audience, represents also a threat.
It's both a threat and an opportunity,
but we have to look at both sides.
So this is why we are working on
achieving crypto agility and creating
realistic and practical post quantum
crypto strategy so that we can keep
our data and our customers data and
our communication channels secure.
But in order to prepare to Y2Q,
that it's the moment, the day,
when a quantum computing be,
will be able to break encryption.
So that's the first thing.
And here we are, we do this.
Internally for ourselves, but we also
have have been working with different
companies in the financial sector to
guide them and to also share our our
advice, but also hear about their thoughts
on how to implement these post quantum
cryptography strategy so that ideally
all the companies are quantum secure.
The second thing that we're doing also
with clients and also internally is we
have a whole portfolio of analytical
tools and to provide financial
intelligence and ultimately help
business leaders make better decisions.
And So what we are doing is understanding
where quantum can disrupt our
products, which means inside of our
products we have underlying there
are a lot of problems and therefore
some of these problems potentially.
could be enhanced or could be solved
more efficiently with quantum.
Not all of them, not always quantum will
be the answer and sometimes the quantum
advantage that quantum can bring is
not necessary but we have to find these
sweet spots where there's a real business
need for some improvement and where
quantum can Provide that improvement.
So for that, we're working with the
classical teams that have products for
the banking sector for the insurance
sector and trying to analyze what are the
real business needs and the bottlenecks
potentially where quantum can help.
So when we find a promising use case,
whether for a client or for one of our
operational units what we do is we focus
in that use case and we do proof of
concept after this use case discovery and
benchmark different quantum techniques
in order to understand whether that
will be the most promising one, maybe
in the future, maybe the technology
is not available there, but We need to
understand if it's promising or if we
should explore some other technique.
And then the third one where we're also
engaging, not just with clients, but
also with academia open communities,
as I told you in my career path, this
kind of communities were very relevant.
So we are engaging with the
community, with the technical
community around quantum computing
and generating thought leadership.
So this is why we are writing articles
so that we can translate the research
from quantum scientists to the people
working in the financial industry.
We have also done a research
report to understand what's the
status of the preparedness in
the financial services sector.
We are working in designing training
materials, also collaborating with
student groups or universities that
have a program that is focusing
quantum science or quantum engineering.
And some we have a lot of examples of
that, but we try to publish everything
we do so that it's open for everyone
to, that want to learn about it.
And we also try to engage in
the activities that are related
to these student communities or
master programs at university.
Dan: so many fascinating topics.
So I think we're going to dive into
a few of them during this podcast.
Why don't we start with the report?
I understand that Moody's analytics
released a report, quantum computing and
financial services business leaders guide.
Could you give us a quick
walkthrough of what that is?
And we'll put the link in the show
notes for people to go and read
this, but what's the purpose of
this report and what's the kind of
executive summary that it concludes?
I know that there's some various
contributors from different
customers or companies out there.
And you also talk about
classical bottlenecks and so on.
So it'd be great to
just hear through that.
Carmen: Okay.
Thank you so much for that question, Dan.
So actually, this was a very interesting
report because what we had seen is that
all previous reports that were out there
that focus in The status of quantum
computing in the financial industry, the
contributors or the respondents always
came from the same group of companies that
we already know are working in quantum
computing, but our approach was different.
We really wanted to have a more
unbiased overview on what is going
on in the financial services sector.
And this is why we interviewed 200 Data
analytics innovation leaders in both
financial services and banking firms.
And we also had six contributors
that contributed to the qualitative
part of the report that are experts
in quantum computing from different
companies like IBM Capgemini's
quantum lab Oracle and so on.
You can find all the
details in the report.
And the findings of the research
were actually very different from all
other reports that we had seen before.
was one of the most impacting Outcomes
or findings of this report were that
almost 87 percent of the financial
firms are under investing in quantum
computing, lacking any kind of budget.
Also, it was clear that there's a
lack of training, because almost Near
three quarters of the respondents
and we're not sure where quantum can
bring a real commercial advantage.
And this means that there is a lot of
work to do and that there is a gap between
the literature and the academic sector
working in in the algorithms and the end
users, the one that have the applications.
They.
really don't know yet where is
the real commercial advantage
and that's something on us.
thing, another interesting finding was
that almost half or a little bit more
than half of the respondents saw quantum
both as a threat and an opportunity,
as a threat in terms of the threat
that it represents to cyber security.
And another interesting fact about
the report was that we also ask
about what were the use cases
that they saw as more promising.
And some of which were risk analysis
cybersecurity, stress testing, synthetic
data generation, and Some that have
to do with machine learning detection
of fraud or money laundering and also
talking about these threat that the
respondents identified in quantum
nevertheless, even if they thought
that it was a threat, 86 percent of
the organizations that we interview
stated that they don't feel they are
ready for a post quantum cybersecurity.
And, 84 percent of those foresee
that they will need to be in
the next two to five years.
I would say that these findings lead, in
summary point out that there is a lack of
training, there is a lack of awareness and
preparedness on how to get quantum ready
and where quantum can provide a quantum
advantage commercial advantage for these
companies, so this gives gives us some
homework so we have to keep doing thought
leadership, we have to work on training
materials aimed at People working in the
financial services sector and we also
need to work with our clients in order
to help them design a quantum strategy.
Dan: Very nice.
Yeah.
My understanding, and it's not a very
well educated one on the financial
sector is that there's a lot of it's
based a lot around risk management
and I suspect in the backend
there's a huge amount of complicated
algorithms and systems which are doing
a lot of this in an automated way.
I know Moody's analytics has a great
focus on risk management and you provide
risk management services to financial
sector and other sectors in the industry.
Specifically with risk management,
how does quantum apply?
Is it one of the use cases or does
it, is it more of a broader topic?
Does it include the business risk as
well as financial risk and another
thing, operational risk and so on?
Carmen: Yeah, that's a good question.
And when we're talking about risk
management, there are many problems
underneath that you need to solve in
order to be able to manage all the
kinds of risk that a business may face.
And in the end what you want
is an aggregated view of risk.
So you don't want to look at each kind
of risk separately, but you want to have
You have going to have an aggregated
view of that and how it's kind of risk
influence is influences each other.
So some of actually it's not that
different that the three categories
of problems that we're looking at
are again the same that are that
I talk about in the beginning.
So there's a lot of modeling and
simulation tasks that we may want
to speed up or do more accurately.
There are a lot of optimization
problems underneath as well.
And There is also machine learning
techniques that could potentially
be enhanced with some quantum
subroutines in the algorithms.
So I would say that the problem
categories are the same.
It's just about the concrete instance
of the problem that you look at.
Steve: As you were speaking,
I thought about something.
So when it comes to looking at
clients problems and thinking about
how to work with the client, I can
imagine that it's not, this type of
consulting and looking at these new
problems or looking at old problems in
a new way, using quantum is it's not
really a structure of how to do that.
So I can imagine.
Clients don't really know what they
want yet when it comes to quantum.
Is that what you see as well, or they come
with a specific problem to solve already?
Or how does the interaction usually go?
Carmen: I would say that we have a lot
to learn from our clients, and actually
they are the ones who can give us advice
on what are the real business needs.
So the same way we need researchers that
are experts in quantum algorithms, trying
to speed up certain computational tasks,
we also need these clients that day to day
applying the algorithms and using them to
get information that is useful , for them.
So I would say that the role of
the clients here would be more of
giving us information on where are
the actual bottlenecks and where
are the actual business needs.
So that we, that can point us
to real world applications that
are relevant to the business.
So probably.
There I would see, I would say that it's
on us to make the right questions, but the
answers, it's the clients that have them.
Steve: Makes sense.
Yeah, for me, I'm just thinking this kind
of business model is so unique and so new.
I don't know if there's any algorithm
to come from client problem to solution.
It seems like each client has
its own needs and own solution.
That's unique.
Okay.
. And you said you've come from a
point where you've had a lot of
involvement in community development
and organizing these events.
Do you still do that today or is that
a big part of your day to day task?
Carmen: Yeah, I could say that once
you become part of these communities,
you never really leave them.
And even though I started in Spain,
here I also try to be close to
academia and I'm based in Zurich.
And one of the institutions that we
collaborate with, it's the master's
in quantum engineering from ETH and
actually there's a very active student
association that it's called the
quantum engineering commission and
We have been collaborating with them.
So for instance, we did a hackathon with
them this year where we were one of the
sponsors and we proposed a challenge,
but also we shared with them the
positions that we have as internships.
And we ended up hiring as a summer
intern, one of the students of the master.
So I would say that,
yeah, I'm still involved.
Actually, I'm officially still
an organizer of a couple of
communities in Spain, the one in
Madrid and the one in Barcelona.
And from time to time, we also we try
to think about what are the activities
that we potentially could do with them.
Talking about Barcelona, another
thing that we did this year was
a hackathon as well, and there
was IBM the Institute of Photonic
Sciences from Catalonia, Barcelona
Supercomputing Center, and and also us.
So there were these four challenges.
So I think that looking at this kind of
collaboration . You can see like the value
these communities can bring because for
us, it's a talent pool where we can stay
close to the talent of the future and
eventually Maybe even hire people that
we know from those events, but also it's
a way to foster open collaboration and
a group that, for example, in Barcelona,
it started as a group of volunteers
doing open talks for people who wanted
to learn about quantum science ended up
three years later in events that involve
Some of the most relevant companies want
working in quantum computing, and I think
that going to an event where you are
going to meet researchers from IBM people
quants from Moody's that will tell you
how they are playing quantum computing
researchers from supercomputing center
also working in developing algorithms.
And.
People from an institute like the
Institute of Photonic Sciences of
Catalonia, and it's such a unique event
and it's such a unique group of people
working together that couldn't happen.
If there wasn't like a live
community of people that are
enthusiastic about quantum computing.
So this is why we think
it's important to be there.
And also, I already mentioned that
we cannot just wait for companies
working on quantum computing to
develop the use cases that are
interesting to us and to our clients
if we don't ensure that we are there.
So the role of academia and researchers
is ensure that progress is based
on a strong foundation and our role
is to ensure that progress is made
on use cases of real world value.
So I would say it's very important
to, to be there and we have clear
what our role is and the value that
we can bring to those collaborations.
Steve: Yeah, I agree.
I've been to a couple of those
hackathons myself and I always see
a huge turnout and a lot of people
excited about developing something new.
I think most people come without
the experience and they learn a
lot in the two or three days that
the hackathons usually run for.
And I think, yeah, you're right.
It's a first introduction to.
Quantum computing, and then they have the
spark that changes their whole trajectory.
I think most of the people working
in quantum, I've had that experience
themselves your experience as well.
And I've had the same moment at some point
where you just see this quantum computing
and now it's a different mentality,
different career path that you want.
And I think without these events,
it's hard to get that exposure.
They're really fruitful.
I think.
Dan: Carmen, if using the audience here,
if you'd like to advertise some of the
specific communities in Spain, if I was
a quantum curious Spanish mathematician
or physicist or just anybody in a
different field interested to learn
about these things, where would I go and
how would I how would I get involved?
Carmen: If you are in Spain, in
one of the big cities, then you
have Quantum Madrid in meetup or
Quantum Barcelona in meetup too.
But actually to make it maybe more
international, I will recommend
anyone to check out Qiskit.
org and join their Slack channel,
because the Qiskit community is
a community that is super active.
They organize open challenges,
they organize hackathons, they
organize webinars, and everything
is open and free access.
So this is a great way to
meet other people enthusiastic
about quantum computing.
And also I would say that the Google
developer groups are a great resource.
They are not focusing on a specific
technology usually, but for sure, you
can also get to know people there.
In Germany, I know, push quantum
in France, the lab quantique
that also organized very
exciting events and hackathons.
I already mentioned the quantum
engineering commission that works.
Mostly in Zurich, but also with, if
you are in Switzerland with other
universities from the area and actually
for this hackathon, they invited
people from all over the world.
So there were people from
more than 25 universities.
So yes, they try to stay tuned and
follow these active communities.
And eventually you might get an
opportunity to collaborate or to join.
Dan: So Carmen, . We were discussing
before the podcast about a new product,
a new platform that you're, you've got on
the market, or you're going to be bringing
onto the market and launching very soon.
This is a great opportunity to hear
a little bit about what you've been
up to in terms of benchmarking.
for algorithms and applications
in the finance sector.
So give us a view on that because it
sounds like it's something I haven't seen
anybody else doing at this point in time.
Carmen: Yeah, indeed, Dan.
This is one of our strong focus, and
we will have an announcement very soon.
What we have decided is that looking at
the findings of the report that we saw,
and how there was a lack of understanding
of the commercial value that quantum can
bring, and also the integration step was
not clear because the learning curve is
steep Companies need to understand how to
adapt their problems and which ones they
can solve with quantum computing, and
also they need to understand whether they
can actually bring on a business value.
So that's why benchmarking is a crucial
step in the process of evaluating which is
the way to go, which is the Best technique
out there to solve a certain problem.
So this is why we're working in building a
product that is a platform that it's going
to be a quantum for finance platform.
And what we will do is enable access to
quantum and quantum inspired technologies.
covering the full stack.
The idea is that this platform
will be problem centric.
So this means that you will define
everything around the problem.
So let's say that you have decided that
portfolio optimization is an important
problem that you want to focus on and
you want to understand which is the
best technique available or the most
promising quantum technique to solve it.
But you also want to benchmark that
solution to what you have today or
what is the state of the art today.
So that, in that case, what you
will do is define different solvers.
One that is the state of
the art classical solver.
And then they also input some
quantum inspired or quantum solvers
that you want to benchmark against.
And You could run the execution of solving
the same problem coming up with the
optimal combination of weights for the for
the assets in your portfolio, for example,
and you will run those independently for
all of the versions of the solver that
you want for the classical one for the
quantum one that will be executed in
a quantum computer and for the quantum
inspired one that will be executed in
a classical high performance machine,
maybe also in some nodes in the cloud.
And what you would get is a graph
comparing with the metrics that
are relevant to that concrete
problem comparing all the solvers.
So this is solving the
integration problem.
Also it enables you with
benchmarking capabilities.
It also solves the problem of
accessing multiple quantum hardware
providers because the platform
has access to many of them.
And we are taking care of enabling that.
And We still cannot announce
who our partner is gonna be.
This will be coming soon.
We are gonna do a PR about it.
But you will have access to some of the
state-of-the art quantum, one quantum
inspired algorithms that are provided
prior algorithm provider that is
gonna be our partner for this project.
Dan: So yeah, the thought that goes
through my mind is if I was a customer
and I had a particular problem that I was
already trying to solve in a classical
way, or I was solving in a classical way
and it was taking an inordinate amount
of cost or time how would I then go about
using this platform to test it on the
quantum systems that you are enabling?
Does it require a services
wrap with Moody's?
Or is there some way that it refactors?
I guess it's not automatic, but
there'd have to be some delicate
refactoring of some kind, right?
Carmen: The effort for now is that the
platform is gonna be a problem centric.
So ideally customers, what they will
bring is the problems that they are
interested to work in, and we will provide
the algorithms that they can compare.
So we see how it goes with these first
marketplace of problems and algorithms.
And then if our client is interested
in doing something more than we could
engage . And help them do use case
discovery and maybe add more capabilities.
Dan: Very cool.
Thank you.
Hey, I'm gonna go back to some
of your points you mentioned
around financial use cases.
So I watched this fascinating webinar
with Mastercard actually, called
the Quantum Advantage, and they were
focusing on different use cases.
I wondered if I could just bounce a
few off you and get your perspective.
I know you mentioned fraud detection,
and they also mentioned offer allocation.
, net settlement challenges hidden
flow discovery for identifying
stuff moving between different
cryptocurrencies and things.
And settlements across border and so on.
I know that Moody analytics is
focusing on a few different ones.
One that I've seen is strategic
asset allocation or perhaps
optimization of assets.
These use cases, they make sense to me
on what they're doing with the financial
data and assets at a high level.
But the actual.
Mystery behind how they operate
and how quantum is relevant
is a bit of a mystery for me.
So if you could talk
to that would be great.
Carmen: Yes.
Thank you so much for your question.
So actually, portfolio optimization
is one of the use cases that
we are focusing on right now.
The way we're approaching it is . You
may know that there are different
paradigms in quantum computing.
We are exploring where we can
get advantage in all of them.
The first one would be
quantum inspired techniques.
And actually, this is a very
interesting paradigm, because
there is no quantum computer here.
These are techniques that are
inspired by how quantum devices work.
And...
Getting inspired by that, you
design and develop new algorithms.
So these were techniques that have
been there for a long time for the
study of quantum many body physics, but
actually thanks to quantum computing.
pushing the limits of computation.
People that were working in
classical computations have also
thought of more clever classical
ways of solving specific problems.
And one of one of the techniques,
or one of the fields inside quantum
inspired techniques is tensor networks.
And actually, we also had an
intern this summer with us that
was an expert in tensor networks.
She came from the Max Planck Institute
in Munich and she was working in
adapting the the problem of portfolio
optimization or a strategic asset
allocation to tensor networks.
So the first thing you have to
do is translate your optimization
problem where you have an objective
function with some constraints.
You have to translate
that into a Hamiltonian.
Afterwards, you need to write
the Hamiltonian as a tensor
network, and then you are in a...
new kind of world where you have a
lot of cool tools that you can try
out and study the convergence of
different methods, understanding
how you can maybe optimize them.
So this is a whole direction of
research that we are analyzing and
One of the promises that these methods
have is with classical problems, one
of the main problems that we have with
classical algorithms, for optimization,
is that many times we get stuck
in local minima or local maximums.
This means that you are not finding the
global optimal solution of the problem.
But if you're using tensor
networks, there are certain methods
that can ensure convergence.
. So this is one of the promises
that we are researching about.
And the other one would be speeding up the
process of finding the optimal solution.
But then this is not the only paradigm
that we have in quantum computing.
So when you are saying that you are
researching how to enhance your algorithms
or how to substitute your algorithm
with a quantum technique, You need
to look at more than one technique.
So quantum inspire is the first
part of them, but then we're
also trying to analyze whether a
quantum annealer can help here.
And eventually we will also
look at variational algorithms.
For the digital quantum computer
paradigm, but right now we are in the
first stage where we are researching
about tensor networks and we have
already started with quantum annealers.
And in the future, we will also consider
variational and variational algorithms.
Steve: Yeah, very interesting.
I think it's such a novel field and
applying machine learning in the
quantum regime to classical problems
is a, it's a wide open field.
But I noticed that one of the core
parts of doing financial predictions
or maybe financial estimations
relies on Monte Carlo simulations.
That's one of the tools that is
used at least in classical sense.
And I know there's a quantum
analog to Monte Carlo simulation,
a quantum Monte Carlo.
And I noticed there's a trend now that
there was an algorithm released maybe
two years ago, three years ago, about a
low depth quantum amplitude estimation
that now enables performing a Monte
Carlo simulation on real devices.
Is that something, is that one of
the reasons why it's an important
problem or what do you think about
quantum Monte Carlo in general?
Is it going to be
something we can perform?
Carmen: Yeah, exactly.
You were right.
Actually Monte Carlo simulation is one
of the tools of the methods that many of
our products use underneath and that is
relevant for many business applications.
And it is a bottleneck in many cases.
And the good news is that there
is a quantum version that promises
a quadratic speedup versus the
classical version so this is why we
are also looking at this use case.
We think it's a use case more for the
long term, but Since it is very relevant
to us we cannot lose sight of it.
And we have to understand what
are the different techniques that
are proposed in the literature.
And originally, the first algorithm
that you can apply in order to speed up
Quantum Monte Carlo was the canonical
version of Quantum Amplitude estimation.
But these first version of the algorithm
resulted in very, in, very deep circuits.
which is bad because the more
that we have means that the
more operations we have to do.
And right now we have
a problem with noise.
And the, one of the things that
researchers working in quantum
algorithms are trying to do is to
make circuits as shallow as possible.
And so this is why throughout
the years there have been
different proposals of the same.
algorithm, but new versions like,
for example, IBM's iterative quantum
amplitude estimation or a maximum
likelihood quantum amplitude estimation.
And more recently Stephen Herbert
from Quantinium proposed a New
method that is called Fourier
quantum Monte Carlo integration.
And what it does is that one of
the steps that results in many
gates, it's abstracted from the,
or taken out of the circuit.
And what they do is like they serve
that part classically in a clever way.
And what they do is they result
in many shallower circuits
that they can run in parallel.
And therefore they are aiming to, to come
up with a version of the algorithm that
could be executed More in the near term.
But again, we think this is a use
case that will only be feasible in
the long term because probably it
will need a error mitigation or error
correction, but it's so relevant
that we are still exploring it.
And actually the challenge
that we proposed in ETH is
related to this problem.
So we gave them the paper of Stephen
Herbert and other papers and we let them
compare all of the methods and choose
which one was the most relevant one.
And this also, this kind of strategy of
breaking up an algorithm in tasks that
can be done in parallel is also one
of the things that we need to look at.
And if we see that If we look at the
different players we see that they
are trying to solve the orchestration
of the different tasks in a workload.
So some examples of companies working on
that are NVIDIA with NVIDIA CUDA Quantum
Zapata Computing with their orchestra
framework, or for example, Covalent.
So what they have realized is that
quantum computers are Just another
accelerator in the data center,
and therefore, we need an efficient
software to orchestrate the tasks
that we send in parallel to different
accelerators, but also to different
quantum computers, and then how to
gather again the data in order to derive
the results that we are interested in.
And I will say that the Stephen
Herbert's Fourier Quantum Monte
Carlo integration algorithm.
It's a great example of this kind of
algorithm that can be parallelized.
Steve: Yeah, I think this was
a topic that I just noticed is
starting to catch traction is
parallelization of quantum algorithms.
Because if you have many quantum
computers, for example, IBM I'm sure
has maybe 10 to 15 in a single room.
Why not make use of them all at the same
time and execute different parts of the
algorithm at once instead of trying to
build a huge quantum computer that can
do the larger scale circuit executions.
Yeah, very interesting.
Dan: It's fascinating
to hear you both talk.
Ultimately, you're pushing the boundaries
here of where we are first of all with
the software and your algorithms needing
something closer to fault tolerance.
And then Stephen, you're talking there
about going back to one of our previous
podcasts, talking about the need
for networking between and somehow
parallelizing, somehow coordinating.
Running algorithms on multiple
machines, but let me just take a
step back and zoom out for a second.
There was one of the use case
that I saw that dumbfounded me.
If we can achieve this, then it I'm
just amazed to know how it worked
and that was recession prediction.
We all live in a world where we either
suffer or benefit from the macroeconomic
kind of events and also the economic
environment in our countries and so on.
But how would you predict a recession?
Of course there are , various different
signs and signals in different parts
of the whole financial environment.
But I guess are we talking
here about another modeling
set of modeling techniques?
Is that really what it is?
I'm looking for a pattern across
all of the different sets of signals
that we can see from the market.
Carmen: So this is a very good question
and actually is one I would say is the
first piece of research that we published.
We did this work together with Rigetti
Computing and Imperial College, and
this is a particularly challenging task
because when Economic recessions are
rare events, and also there are many
variables that can influence a recession.
Because they are rare events, the data
that we have is usually incomplete, it's
noisy, and for some countries, we even
have ... little or close to no data.
This is why , it is such a challenging
task because most statistical
models, they rely on examples of
past events in order to predict
what's going to happen in the future.
What we focus on what they are research
focused on was comparing the ability
of quantum and classical prediction
methods in this particular task.
So in this case, the role of quantum
computing was to augment some machine
learning processes that are used.
And what we found out is that the
a method that it's called quantum
based signature kernel methods deals
some promising initial results in
terms of the accuracy and the early
recession warning capabilities.
So this also indicates that
They have potential not only for
predicting economic recessions, but
also for a time series modeling.
So right now we have continued
collaborating with Rigetti.
The further work that we're focusing on
is going to assess the effectiveness or in
dealing with problems with a higher number
of dimensions of features, and also to
continue to investigate some improvements
in the quantum model, the optimization of
the execution times, and even looking at
some quantum error mitigation techniques.
So this is what is going on,
and we hope to generate further
progress and eventually publish
the results of our research.
I have to say that here.
Our main role has been providing
the real use case and the quantum
techniques that we have tried out
a have been developed by Rigetti
Steve: Yeah.
So there's different areas in
where quantum computing can apply.
. There's a risk management that
I guess is the focus of Moody's.
Do you have any other directions in
which you think quantum will make impact?
I guess the question is a bit general, but
it's and I know that there's things like
chemistry, a quantum simulation, , but
maybe these are not something of interest
to Moody's or to you at the moment, but do
you think there's something coming where
quantum will make the biggest impact.
Carmen: So yeah, you already gave
the answer that I have in my mind
because of all the literature and all
the recent publications the first use
case where we expect to see quantum
advantage, it's in the original use
case that Feynman proposed, right?
Like understanding nature better.
So simulating computers to
simulate an actual quantum system.
But we had to focus and on quantum finance
where the systems that we are simulating
are not quantum one of the techniques that
we think could be could bring a benefit in
the short term are these quantum inspired
techniques that we were talking about.
So this is why we are
pushing hard on this.
As I said, we had our first
person that is an expert in the
field and we are planning to
keep pushing that exploration of.
Applications of tensor
networks to finance.
Steve: Yeah, I think it's all
about exploring at this stage.
There's so many things that we didn't
discover, especially classical quantum
hybrid algorithms, how to leverage a
little bit of quantum, a little bit
of classical to solve problems that
we can solve maybe earlier than later.
Use a little bit of NISQ, a
little bit of GPU, who knows.
So just massaging these problems to a form
that we can apply the techniques we know.
To the problems that are important
to people I guess that what comes
to that is, is a barrier, right?
This is not an easy thing to solve and
therefore there's definitely a lot of
hurdles to overcome on the path to,
using quantum computers for applications.
Then my question is therefore, what do
you see is the biggest challenge to solve
before we can start deploying commercial
applications to, to use quantum computers?
Carmen: So one of the challenges that was
actually found in the report that we did
was this lack of understanding of where
quantum can bring a commercial advantage.
And I would say that it's not an easy
task to do this use case discovery.
And once you have
identified the sweet spots.
That are promising.
You need to map your problem
to to a quantum representation.
So you have to transform it in a way that
you can apply one of these techniques.
So for that, you need the expertise,
and the learning . curve is steep.
The talent pool is scarce.
I think one of the challenges that we have
is educating the workforce of tomorrow.
And there are many universities that
are doing a great job on that, creating
specialized master programs, and also
engaging with the industry so that they
can have some subjects where students
are exposed to the real world problems.
This is definitely one of the
challenges, the lack of education.
Another one could be what we already
discussed solving the problem of how
to integrate a quantum with other
accelerators in the data center and how to
orchestrate that in a clever way so that
you don't get extra latency from that.
And in terms of the technology itself,
I would say we are waiting for the
hardware to scale for better error
mitigation techniques to come and
eventually error correction, but there
have been some recent breakthroughs
in the field that are promising.
So we are hoping that this will
become a reality in the years to come.
Dan: Thank you very much.
I think we're going to wrap it up
there, but I wanted to say first of
all that it's fascinating to get a
view from a particular application
. In this case, the financial space.
I think it sheds a different light onto.
The applicability of quantum for me.
And I hope it does the
same for our listeners.
And in fact, anybody who's listening
this far, if you think this is a an
effective approach for us on the quantum
divide, then feel free to recommend other
industries propose if you think this is
a good method, then then, drop us a note.
It'd be interesting to hear
we verticals in the future.
In the meantime, let me
come back to you, Carmen.
Thank you very much.
Really appreciate you giving us that
technical depth and a great conversation.
And I wish you the best of luck at
Moody's and with the projects and
initiatives you're working on at the
moment, both inside the company and
outside with the communities as well.
Carmen: Thank you so much Dan and Stephen.
I really had a lot of fun being
here today and it was it was
a great way to start the day.
Dan: Superb.
Thank you.
I'd like to take this moment to thank
you for listening to the podcast.
Quantum networking is such a broad domain
especially considering the breadth of
quantum physics and quantum computing all
as an undercurrent easily to get sucked
into So much is still in the research
realm which can make it really tough for
a curious it guy to know where to start.
So hit subscribe or follow me on your
podcast platform and I'll do my best
to bring you more prevalent topics
in the world of quantum networking.
Spread the word.
It would really help us out.
Creators and Guests
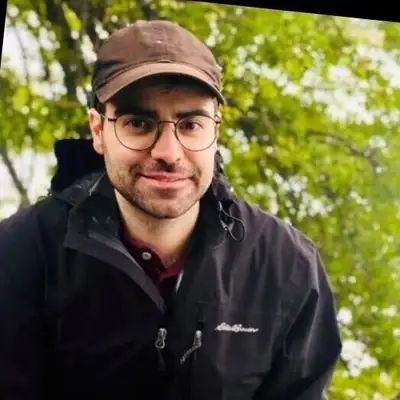
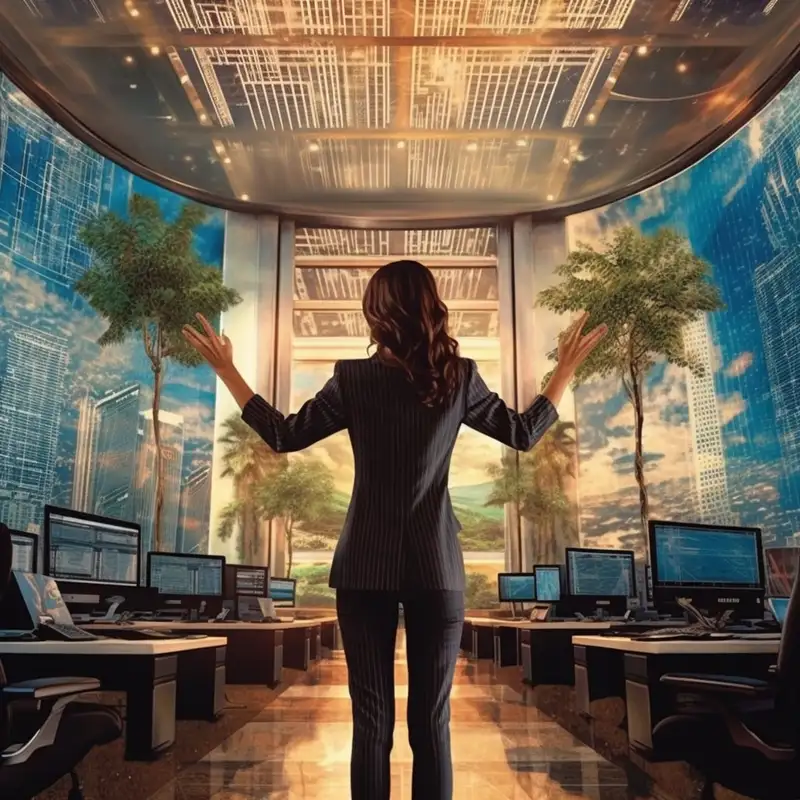