Quantum Sensing in Munich, with Kevin Berghoff, QuantumDiamonds.
Dan: Hello there.
Welcome back.
Thank you for tuning in once again.
This week, I am joined by a
gentleman called Kevin Berghoff.
Kevin is the CEO and co-founder.
Of quantum diamonds.
Which interestingly is a
quantum sensing startup.
Slash scale-up yeah.
Based in Munich, Germany.
, their mission is to make
the invisible visible.
So they are developing or
have developed atom sized.
Quantum sensors using nitrogen
vacancies in diamonds.
, They, , are used to detect magnetic
fields, and their mission is to build
a global sensing platform that enables
advancements across a variety of fields
from semiconductor manufacturing industry.
To biotechnology and chemistry.
, that's my intro.
So let's get into it.
Thank you.
alright well thank you Kevin
for joining us today quantum
networking Weâre taking a bit of
a dive into the sensing world here.
Dan: How did you get into quantum
and what was your path, your
career path before getting into
uh, working for Quantum Diamonds?
It'll be really interesting to hear.
I know, I think you have a
slightly different, , route
in compared to some others.
Kevin: Yeah.
Thank you.
Thank you so much for inviting me.
So I think what is very interesting
is that I'm the only non
quantum physicist in our team.
So I actually have a pure business
background, so studied international
management first in Cologne in Germany,
and then did a master's in Lisbon
in Portugal and in Milan in Italy.
Uh, Afterwards or as part of the
studies did a lot of internships
in various fields and then as many
business guys, I ended up in consulting.
So I worked for McKinsey for three
years, did a lot of projects in different
industries, mainly in the private
equity fields, a lot of due diligences,
companies being bought, being acquired.
And then actually as part of the LEAF
program at McKinsey started a PhD at
the Technical University of Munich
also at the business field, but then
was actually involved in quite a lot
of courses at the university where
also many technical PhDs were present.
And there I actually met Fleming, our CTO,
or now CTO at that time, a PhD student
at the Technical University of Munich.
And he told me he's working
on diamonds and quantum.
So I didn't know anything about it, right?
So I didn't have a lot
of quantum experience.
I know that, for example, McKinsey
did also some work in terms of
technology, innovation and quantum
monitor how the landscape evolves.
but never heard of quantum sensing before.
And then at some point there was a
large German conglomerate, Bosch,
that actually approached the research
group and asked if they could
buy one of these quantum sensors.
And I was a bit surprised because I know
a bit about the corporate world, so it
usually is very uncommon that a large
conglomerate reaches out to a university.
research group, right?
So this was the first point where I
thought, okay, maybe there's something
behind it, like a business case.
And then we jointly looked at it.
So I looked mostly from the business
side, the application side, and Fleming
looked a lot at the technical feasibility.
So we try to understand the different use
cases where these sensors can be applied.
And then at some point
found very interesting use
cases, started the company.
And I think we go into the details
about the company in a second.
Dan: Yeah it's fascinating
to hear your perspective
often that, the these things start
with a chat with a colleague, or a
combining of different perspectives.
So tell us a bit about the company.
What's the size, the scale of,
of the company at the moment and
what are your goals going forward?
I think you've recently had
some funding as well, right?
So if you could talk us
through that'd be good.
Kevin: Yup yup
so we started the company one and a half
years ago, so pretty new company still
finalized a seed funding round in December
four months ago in total 8 million, a
combination of private and public capital.
from leading VC investors, early bird from
Germany, IQ Capital from the UK, but then
also a couple of smaller VCs joining first
momentum onsite ventures from Austria.
So a lot of investors that not only
bring deep financial pockets, but
also experience in at least a couple
of the verticals we are targeting.
We now have established our own
kind of headquarter combination of
yeah, lab infrastructure and office.
So now we completely left the
university setting and are 15 people.
I'm the only non quantum physicist,
so highly technical team.
And yeah, we are about to
launch the first product.
You can think of it as a microscope
where you can do detailed
analysis wherever you want to.
measure physical properties very
precisely and are still continue to
hire, continue to onboard new people
from this field and enlarging the team.
That's what would be the
milestones I'd say for this year.
Steve: And so you founded the company
in Munich, were able to leverage some
of the government initiatives in Germany
for getting your company off the ground.
Kevin: So we actually started
before we started the company.
So there's one one, one
funding scheme called exists.
It's like a founder scholarship.
So basically it pays three base
salaries for for founders for 12 months.
And this is what we, where we apply to it.
It's intended to bring deep tech
to entrepreneurial activities.
And that's actually what we got.
So Christina Fleming, the
two PhDs and me for a year to
prepare founding the company.
And afterwards we also receive funding
as part of this 8 million seed round
from from the EIC the EIC accelerator
program that you might know of.
But then also there's a state level
funding from the Bavarian government.
It's called the Munich Quantum Valley.
And there we could also leverage 1.
5 million.
That's highly attractive from a
founder's perspective, because in the
end it's non dilutive and of course,
also attractive to private investors.
If we're talking about quantum and still
yeah, deep tech and requires deep pockets.
Dan: You both went to
TU Munich, didn't you?
Do you think you guys might
have crossed paths unwittingly
at some point in the past?
Steve: Perhaps I was
there from 2015 to 2021.
Yeah,
Kevin: it's a huge university, right?
You always in hindsight realize that
you should have met someone, but
you never actually talked to them.
Steve: I wasn't, I was in math
first and then I moved to the
city electrical engineering.
I think that if you're in the
physics group, I know the back of
the campus in Goshing, that's where
all the quantum stuff's going on,
keep it in, maybe one, one time.
Dan: So Kevin, you mentioned
Bosch earlier on, are you
still working with them at all?
Kevin: Yeah, so we're, we still, I mean,
it's a kind of close community, right?
So there's no, A new entrant that somebody
that comes at one point and then leaves.
So it's a very close net, I would say.
And yeah, we're still in contact.
So they are focusing mainly
on the medical field, but also
pretty exciting technology.
And yeah, the community is small,
so we keep the exchange ongoing.
Dan: so which part of Bosch is it?
You said the medical use case.
Is that for Magnetoencephalography
Kevin: Yeah.
Yeah.
They don't review too much, but this is
something that they actively look at.
And I think it's still part
of the corporate research.
So I think they still figure out where
to put it in the big corporate structure.
But I think it's under the
corporate research right now.
Dan: yeah.
I mean it's, I know Bosch a little bit
and it's no surprise that they would've
reaching out to universities in Germany.
They're like a innovation
engine, uh, really connected
to all parts of the country.
Kevin: Yeah, they were connected.
But at the same time, it's still, I
would say a different game founding
within Bosch with all the resources and
founding a kind of standalone company.
It has pros and cons.
I would say the flexibility and the
speed is something that probably you can,
better leverage if you're a standalone
company, but it's still like a great
corporate and great infrastructure
and resources that they have.
Steve: I think it's also not as uncommon
that the industry companies come to
the universities because, especially
with quantum, most particular with
quantum, because quantum is actually
behind in industry, I think, compared
to academics, even in lab settings.
So these bulk optics, bulk
experimental designs is cutting edge.
It's as far as it goes.
So they don't have as
much as the university.
So I think it's
Kevin: Yeah, true.
We see the same if we are now in the
hiring part and try to get experienced
talent from the industry, there's no
experienced talent in the industry, right?
People come from university, and if
they have three to five years PhD
experience in the field, That's the
most advanced or the most experienced
talent profile you can actually get.
I think this connects well to
to corporates reaching out
to research groups directly.
Dan: Okay, let's move on to
the products that you have.
Give us a bit of a deep dive on
what you've got on the truck,
so to speak, as they say.
But actually, how you've got
to this point in time, right?
Because, and I would expect there's
been some kind of iteration of
development as you've gone through.
So it would be interesting to hear that.
Kevin: Yep.
So we started with the hypothesis that
you can sell these quantum sensors to
many, many different industries, right?
So in the end, if you think about
a magnetic field sensor, it can
be used in whatever industry needs
magnetic field sensing, and if you
look closer, it's biology, chemistry.
Semiconductor manufacturing, battery,
almost all materials you can investigate
using magnetic field sensing.
So we thought in a couple of years, there
might be many industries needing these
quantum sensors and let's become the one
stop shop where everyone buys, right?
So the hypothesis was.
Bosch is developing it for healthcare
Siemens is developing it for medical
imaging, BMW might use it for their cars.
So the idea was that we really are
the intermediary that finishes these
quantum sensors and makes them reliably.
So this is something
that we already offer.
We have products in the market where you
can buy ready to be used quantum sensors.
That's where we are right now.
But then talking to these end users,
we also realized the big value lies
in integrating these sensors into
a device that you actually use.
So we realized also talking to the
automotive industry, they would never
actually buy these these quantum
sensors in themselves, but rather
have some final use that they can
just plug and play into their existing
manufacturing line into the technologies.
And then we came across the
need to actually build a, an
integrated imaging system.
So not only.
The core itself, let's call it lens
maybe or an objective, but integrated
and then not only provide the hardware,
but also the software that comes with it.
So we started with the ready to be used
quantum sensors, but now granularly
moved into integrating it into a device
that you can think of as a microscope
where you can then do ultra precise
measurements in a variety of fields.
Dan: Cool.
So the products that you have are
nitrogen vacancies in diamonds, right?
Could you maybe walk through how
they work and how you make them?
Kevin: Yeah, sure.
Exactly as you said, we use these
vacancies in synthetic diamond material.
That's particular or one, one
highly studied quantum sensor.
And what we do in the end is
we buy synthetic diamonds.
material.
So that's commonly available.
It's used in other verticals and drilling.
These synthetic diamonds are
used for a longer period.
And then we, we actually change the
diamond structure on an atomic level.
So we shoot in nitrogen atoms into the
carbon lattice, and then we actually take
out the neighboring atom so that they
form a so called nitrogen vacancy center.
And that's the core
component of our sensor.
So it's only, two atoms in size,
you have the nitrogen atom, you
have the neighboring vacancy.
And what these two atoms
have is a so called spin.
So in the end they have a spin
that we can use for measurement.
If you're not a quantum physicist, I
like to call it a kind of compass needle.
So think about a compass needle that
reacts to the magnetic fields that are
exposed to, and the core diamond that
we have is in terms of dimensions,
roughly four by four million millimeters.
And it contains over 10 billion of
these little spins, these compass
needles that we can use to basically
measure magnetic fields very precisely.
Dan: So if your product is just
two atoms wide or as small as two
atoms, you've probably got the
world's smallest product ever.
You should be in the Guinness
Book of Records for that, I think.
Kevin: That's a very good good idea.
I never thought about it, but we
should definitely at least try
to get the challenge out there.
But I think this is a very interesting
point because, In the end, we
think that sensors cannot get any
smaller than these two atoms, right?
We use many of them to average and to
have it on a broader scale, but I think,
but I think in general we a sensor that
cannot really be smaller in the long run.
Steve: One thing that's interesting
is NV centers are used also
for entanglement distribution.
Okay.
This is a sensor.
It's a local device.
Do you have any advantage to purchase?
Perhaps like entangling multiple
of the sensors that are nearby.
I know there's distributed sensing,
which is an idea, but that's also not a
localized thing, but perhaps there's an
advantage if you can control multiple
NV centers at the same time and entangle
them as anything pop up in that domain.
Kevin: I think this is
still on a research level.
So we, I'm not aware of any yeah, industry
use case where we already have that.
So in the end, we don't entangle the,
the spins or the qubits we have but
rather they independently react to the
magnetic field they are exposed to.
But of course there's definitely
something we have in mind when
trying to improve the sensitivity.
Dan: Okay, Kevin, I can imagine
all of the different NV centers
in the diamond material reacting
to uh, call it a global magnetic
field or a change in magnetic field.
How do you take a reading from
all of them at the same time?
Do they produce some kind of is it a
voltage which comes out of the material
or is it more complicated than that?
Kevin: It's actually from
the setup relatively simple.
So what we do is we take the diamond
that contains the little defects.
We hit it, hit this with a green laser.
So that kind of excites all these
uh, spins to an initial stage.
And then we basically expose
it to the magnetic field.
So if you want to test a an integrated
circuit, it produces a magnetic field.
And then these spins, basically
change or react to it.
And what we then do in a second
step is we take a picture of this
synthetic diamond because in the end
the fluorescence the That comes from
putting the green laser at the quantum
sensor it's Defined by the fluorescence
the intensity of the fluorescence.
So To put it very simple, we take
a picture and the intensity of
the red light that is coming from
the diamond can be mapped to the
magnetic field that is directly in
close contact to the diamond sensor.
Dan: Fascinating.
Okay.
So it's a single shot point in time
measurement in a graphical state.
How do you uh, do you monitor
the magnetic field in time?
At all, sometimes.
Do you have a, do you record video
or maybe lots of snapshots and
then analyze them together somehow?
Kevin: Yes, I think what we do
right now is actually we take
multiple pictures per per second.
So the frame rate is relatively
high of the cameras we use.
But I think in the end, what takes a
lot of time is evaluating the data.
So really interpreting it.
So what we could do is
actually a dynamic monitoring.
And this is of course also interesting
for many different use cases.
If you can real time in real time,
look at processes, be it in integrated
circuits and biology chemistry, but
there, we still have to do a lot of
developments right now, we'd rather take.
One shot we evaluate, we do the
post processing and then we take the
second one maybe half an hour later.
But this is something where also
people from the different fields
are excited about to have real
time non destructive imaging of
whatever you want to investigate.
Dan: Nice.
I've learned with just a little
bit of research in magnetometry
that there's a bit of a scale
between resolution and sensitivity.
Where do these NV centers sit?
So what kind of resolution can they
go down to in terms of the area
of the magnetic field that they're
reading and how sensitive are they
in terms of the strength of it?
Kevin: Yeah.
So in general in terms of
resolution, we are on a nano scale.
Yeah.
So in, in the end we're
talking about diffraction.
We are diffraction limited
or we were last year.
Diffraction limit is
roughly 400 nanometers.
There are a couple of innovations
that we came across to, to bring it
down to 200 nanometer resolution.
But what we will on a physics level,
never be able to do is imaging on a
single digit nanometer on the physics
side, but also, and this is the big
struggle we have right now on the on
the processing power and the end we
generate per pixel so much information.
If we do four by four millimeter
imaging on a, nanometer scale.
It's just terabytes of data that are
generated within a fraction of a second.
So a physics limit.
We will not do nanometer imaging,
nanometer scale imaging, but or
single digit nanometer imaging.
But also the core challenge right now
is actually doing all the processing.
Steve: So in that processing stage,
when I think of having to do things
faster, it's usually moved to GPU or
some other type of performance enhanced
or performance specific hardware.
Do you have to leverage any of those
types of hardware for post processing?
Or is it possible to use just traditional
techniques for, a slot of Matrix
multiplication and things like this
that have to go on and to filter the
images, what techniques are there
Kevin: one.
One, one approach we definitely
look at right at the moment is
physics informed machine learning.
So if we are, for example, looking
at multi layer integrated circuits,
we can basically train a machine
learning algorithm, how to separate the
information of the different fields.
So in the semiconductor use case,
For example, you to see per layer
of the chip what would I expect in
terms of in terms of magnetic fields?
And we can use that to go from our camera
image that is naturally just a, a picture.
So no 3d information to then come to
the point where we separate the signal
information of the different layer.
And then we use this approach of
integrating physics rules into
the machine learning algorithms.
That's one We are also looking
at software approaches to do
only selective processing.
So really identifying already at the
camera stage, where do we see some
deviations from the magnetic fields we
would expect to basically cut down on the
data that we capture in the first place.
Steve: Do you think these techniques
can lead to real time analysis or
something has to be invented to,
to make it fast enough for that,
Kevin: No, I think we are
optimistic that what is out there
is already very good, right?
There are even post processing.
approaches to, to increase the resolution.
So there, there are a lot of
approaches from different fields.
I mean, In the end, if you think about
it, we do, image processing, right?
So this is not something we
have to invent from scratch.
In particular, if we do a kind of
comparison of what you would expect versus
what is there, identifying the failures is
relatively straightforward, I would say.
Steve: especially, I mean, the,
the amount of evolution from image
processing, the machine learning field,
I think it's probably been tremendous
in the last five years with all
the computer vision and techniques.
I'm sure there's a lot that can apply.
To sensing uh, image
processing for sensing.
Kevin: Yeah.
As soon as we generated the camera
image, it's not about sensing in
the first place anymore, right?
It's about, Processing the image, maybe
integrating it to the software or the
analytics tools that the respective
customers are using it anyways.
And then it's far away from
conventional sensing analysis.
Dan: So you mentioned the
semiconductor use cases can we just
do a bit of a deep dive into that?
I'm keen to know, , you know,
the details of how it works.
I'm sure our listeners would be
interested, but then also, is
this the primary use case for you?
, and what does that look like
globally for quantum diamonds?
Kevin: Yeah.
Very happy to.
So as I said earlier, so we looked
at all the different verticals and
many of the end users were excited
from an innovation perspective.
So really to see, oh,
there's a new technology.
It has quantum in the name.
So we would have to look into this, right?
There was an, in biology and
chemistry, the same in automotive.
So everyone was open to have
the first technical discussion.
But when we talked to the semiconductor
industry, we really saw that they have a
problem that is worth trying to resolve.
So we we talked to, yeah, a couple of
the big names in the industry and they
told us basically about the problem.
So they said, we have more
complex chip architectures.
We start to put different
layers on top of each other.
We start to put metallization
layers in the devices.
And this leads to the fact that optical
techniques don't really work anymore.
So if you think about classical
microscopes, optical approach,
you cannot investigate more
than the top layer of the chip.
But if you want to make sure that
the more complex architectures work
reliably that you have connected the
different layers, no opens, no shorts
in the layer further down, then you
would need a non destructive way of
testing these integrated circuits.
And there there, the alternatives
are often deconstructive.
So you really have to
cut the chip into pieces.
And then of course you cannot
really, learn from it in operando.
So once you deconstructed the
chip, that doesn't work, of course.
And this is where we started basically to
to understand with the big names in the
industry, how can a solution look like?
And then we are going more into a kind of
imaging device rather than just selling
them one of these quantum sensors.
And yep it's really more complex
architectures, multi, multi layer
there's a trend called backside power
delivery, where you try to put the power
delivery of the chips to the back to
make even more space for the transistors
of the top size, of the top side.
So these are technology advancements.
that require this nanoscale imaging
in a non destructive manner.
Dan: Nice.
Did you allude earlier on to somehow
integrating the quantum The sensing
layer into the product, or is it more
integrated into the manufacturing process
equipment so that it's like a a chip or
a wafer can come across into a sensing
component in the manufacturing floor,
and then it's all sensed in one go, or
are you baking in the nitrogen vacancies
somehow to the actual chips themselves.
Kevin: So I think that's a
very interesting thought.
And I know there are a couple of
papers who do exactly that integrating
a layer of NVs into chips, right?
It has, I think, a huge implication
in terms of the manufacturing process.
So it makes everything, more complex.
We are at the moment rather on a,
I would say, tooling level, right?
So you, we use the NV
centers as the sensing head.
And then you can similarly to, to a
microscope, put one of the samples in,
into this device and then do measurements.
I think this also very
much aligns with how the.
Okay.
The failure analysis labs and the
design verification labs work in the
semiconductor field because you have the
different tools, you have microscopes,
you have emission microscopes, you
have all these different tools and ours
could be placed basically next to it.
And so you say, I have
the conventional tools.
I need the one that is highly sensitive,
non destructive, so you put it next to it.
I think it's easier.
and integrating it into
the chip themselves.
Dan: Yeah, I was just interested
to know if there were benefits in
doing that, you think, at some point.
The papers you mentioned, I
don't think I've read them.
Are these kind of experimental
techniques, if you like?
Research work rather than with
a particular goal in mind.
Kevin: I think it's research based,
but what the big advantage would be
is that you're closer to the signal.
So one challenge that we have and it's
just in the nature of physics, the
further we are away from the signal
source, the more the magnetic field blurs
and the the more the resolution drops.
So if we are a couple of millimeters away
from the signal source, our resolution
drops, whereas if we would have a a, and
the layer in, in all of the different
layers, so to say, and we can basically
approach one of these layers, it's like
in a specific manner, then of course
the resolution would be way higher,
so I think this is the big advantage,
but still from the manufacturing point
of view, it would, I would say be too
complex for like semiconductor fabs.
Dan: Yeah, I guess that's the
inverse cube law, isn't it?
Of magnetic field.
But Ultimately, you're going to be
very close to the source anyway when
you're measuring a chip or a wafer.
You're going to be resting your
sensors directly on the product
or, nanometers away, essentially,
Kevin: Yeah.
Yeah.
So for the first layer, it
always worked, works perfectly.
But if we want to go into the, to the
layer further down, of course, the
standoff distance can become like a couple
of microns, sometimes even millimeters.
And depending on the strength of
the currents, of course, this is
already quite a distance, right?
So it's always a complex combination of
current strength and standoff distance,
but in general, we can be very close.
Dan: high precision kind of activity.
Yeah, so in terms of the competing
technologies, I'm interested to know
your insights there, because I know
that there are different, lots of
different types of magnetic sensors
when it comes to quantum sensing.
Why, first of all, why pick NVs?
Secondly, why pick Diamond?
And is there a stack up against
the other technologies that
would be interesting to share?
Kevin: Yeah, sure.
So the way we think about it is we always
talk about direct alternative technologies
and indirect alternative technologies.
So for the direct alternative
technologies, they also
measure magnetic fields.
So you have GMR sensors, you have squid
sensors that basically also can give you
information about the magnetic fields.
The big disadvantage of GMR
sensors is the low sensitivity.
Yeah.
So it's a very good approach, but the
sensitivity doesn't, it's not really
high enough in particular for the semi
use case and for the squid sensors, you
can, in the end, you also have a non
destructive method, but the resolution
is rather on the micron scale and we
would need to go down to the nano scale.
So this is for direct
alternative technologies.
In terms of indirect alternative
technologies, you have often, particularly
in the semi use case, thermal imaging.
So you use infrared radiation.
It's also same issue, very low resolution.
So we're talking about, maybe microns,
sometimes even only millimeter resolution.
And it's also destructive.
So if you do a destructive measurement
on a, on an integrated circuit,
you cannot use it afterwards.
And similar for for TEM
or SEM measurements.
So you have the electron microscope.
Pick approaches.
And same there.
So if you deconstruct the chip, it's
okay for failure analysis if you want
to do some tests for one, two chips.
But if you think about large scale uh,
yield improvements and high throughput
semiconductor manufacturing, then of
course the deconstructive approaches
are not really feasible for large scale
Dan: It made me laugh using the
terminology deconstructed chip.
It reminded me of that kind of trend in
cooking where they have a deconstructed
tiramisu or something where they have
this separate cream and the separate cake.
Separate chocolate, ultimately
you're destroying it.
It's not a tiramisu anymore.
It's not a semiconducting chip anymore.
It's just a piece of rubbish
that's been broken up.
Kevin: Yeah.
And you have very good approaches
there to go down to like single
digit nanometer resolution, right?
But in the end it's not something
you can use for each and every chip.
Steve: One thing that always comes up
is it's always non trivial to just put
the technology in place that's new.
What type of challenges have you
faced and what challenges are
to still overcome before you get
exactly the product you want?
Kevin: Yeah.
So I would really separate
between the different use cases.
So you can use it or what we
currently look at is design
verification and failure analysis.
So really next to the manufacturing
line of a a chip manufacturer.
So that's there.
We solve the challenge of having
a compact device that kind of
looks like a microscope where
you can put in single samples.
You can do the evaluation.
It doesn't take you a couple of
hours, but rather a couple of minutes
that already works also in the lab.
But the main challenge or where we want
to go as a startup, as a company is
more towards inline quality control.
So you have your lithography machines, you
have the electrical testing, like in the
very end, but to really do inline quality
control help to accelerate the yields.
I think this is something where we
have still significant challenges.
If you think about a very fast
manufacturing line, we need to
increase the size of our sensors.
So four by four millimeter
compared to a wafer.
is yeah, we would have to stitch a
lot, so it would take a lot of time.
And then also in terms of evaluation
time we would be way quicker.
So I think right now we are
in the order of half an hour.
So I think there we just have to go down
the best case to two single digit minutes.
Dan: Is it a matter of just more
compute and more efficient algorithms?
Having just watched the GTC NVIDIA event,
with new GPUs coming out I imagine you've
got a kind of, you're looking at that
already and thinking, how can we enhance
our processing, post processing time,
Kevin: Yeah, that's a very interesting
part because in the end we can use GPUs to
manufacture GPUs more efficiently, right?
Then you have a continuous loop.
But yeah, this is exactly that.
It's about processing power.
But also, right now, it's still an
issue to manufacture these synthetic
diamonds on a bigger scale because
you would always have to have a
electronic grade diamond substrate.
You would have to incorporate these little
NV centers in a kind of homogenous matter.
So there's also on the
hardware engineering side, a
lot of things, but the more.
exciting part for us right now is just
the speed at which these post processing
steps are, like new alternatives come up.
And this is something where we cannot
really estimate also when we talk to our
investors, how much faster can we get?
How much more can we improve the
resolution through post processing?
So, Yeah, it's exciting.
Um, Pretty excited on the software side,
but some, I would say basic homework on
the hardware side is also outstanding.
Dan: I'm interested to know a
little bit about the you're taking
an image of the fluorescence.
So it's, it's not like
you're taking any telemetry.
Kevin: Yep.
So what we have to do in the post
processing is we take the picture.
So we have a 2d picture, I would say.
And then in the post processing,
we have to translate for every
single picture that we that we
have or for every single picture.
I forgot the name.
So we, we take the picture and for
every pixel of the image, we would
have to translate the the fluorescent
intensity to the magnetic field.
And this for each and every pixel
on a four by four millimeter.
If you want to do nano scale
imaging this is a lot of processing.
So there comes the complexity
in terms of software.
Dan: and is it a simple scale between.
Zero to a particular number.
You've got a scale of fluorescence and
it's just mapping for every single pixel.
Kevin: That's the base approach.
We basically sweep the kind of microwave
frequency and then we see some drops.
So there's a lot of like post processing
but in general that's the basic approach.
The easy way to think about it is
that we can map the intensity to the
magnetic field strength per pixel.
Dan: and how about finding defects?
Is it a simple matter of you, you
know, exactly what a perfect chip
would look like, and therefore you
just, you compare your, your output
for all of the individual pixels
against that kind of a blueprint,
or is it more complicated than that?
Kevin: Yes, we are still in the
early stages of this analytics layer
where we proactively even want to
suggest some failure modes we might
expect from a specific design.
But what we do right now is
exactly what you described.
So we have a known good die.
We can do simulations on what we would
expect in terms of magnetic fields.
And then we directly overlay this
with the actual measurements.
And then you can very
nicely see deviations.
It's very clear.
the harder part is then say like analyzing
why and what exactly is happening there
right so you see like deviations in terms
of magnetic fields very clear and also
there are very good papers that show
what other Approaches cannot detect and
then what quantum sensors can detect.
But the, what we want to provide
as a startup is the analytics.
What happened there?
Is it an open?
Is it a short?
Is an issue with the interconnect?
Is it a material defect?
And this is something we learn
over time from just measuring
and measuring thousands of chips.
That's the end state.
But there we are just
in the very beginning.
Steve: One question about the process
when you set up the system to take these
images, do you have to cool it down for
the NV center to be in a quantum state or?
What type of environment is it in?
Kevin: Yeah that's actually one big
advantage that we didn't even discuss yet.
So we can operate at room temperatures, so
there's no need to cool the system down.
So that's a very neat thing because if
you want to, if you have to cool down
in a semiconductor fab the analysis lab,
I think this would be a huge challenge.
And also in terms of clean room
needs, of course, in a clean
room, everything's way Thank you.
easier, but just from the
approach, we can also operate
in non clean room environments.
Steve: Because I would imagine also
in the cooled environment, maybe
the semiconductors don't work the
same way as if they were integrated
into a room temperature system.
Kevin: That's true.
It's very easy to, for us to do these
measurements at room temperature, the
very same temperature that the chips in
the end, which would be operating now.
Dan: So what about
additional use cases, Kevin?
I guess you're already looking
at potential diversification when
it comes to use cases, right?
In which industries you're looking at,
you mentioned a few at the beginning.
Have you decided already on, on
where you want to focus R& D yet?
Or are you still a hundred, I'd
imagine you're still a hundred
percent focused on your semiconducting
manufacturing use case, but yeah.
Keen to hear your thoughts.
Kevin: Yeah, sure.
Also there we separate um, our
thoughts into two categories.
One being the imaging approaches.
So if you think about a microscope
that you might know from biology
class in school, you can your image,
so you basically have a sample holder
and you can put in whatever you want.
So you can put in a blood sample, you can
put in a chemistry probe or any chemistry
material you want to investigate.
So this is the kind of the use cases
that are adjacent to what we do right
now in the quantum sensing field.
There you can do higher
throughput NMR spectroscopy.
So you can look at how do enzymes
grow over time or how do cells
or molecules change dynamically
in real time in the best case.
So it's always taking our imaging
system that we have for the
semiconductor use case and preparing
the samples you want to investigate.
Of course, it comes with challenges,
but it's a very same approach then
to come from the camera, the picture,
the fluorescence to analysis.
So this is pretty straightforward.
And there we are currently discussing
with people from biology and chemistry,
but we didn't decide on a use case.
We have it in mind, but 80, 90 percent
of the focus goes into the semi use case.
The exciting part or something for
the longer term is using these quantum
sensors, but not in an imaging approach,
but use them as a kind of standalone,
so to say, compass needle or like
a sensing system where you don't do
imaging, but you just use one of these
sensors that has a lot of pros and cons,
but there are very cool applications.
You can do navigation and GPS
free GPS denied environments.
And so let's say GPS doesn't work anymore.
We could use the sensor to map
it to the magnetic fields of the
earth, where you are right now, and
then see where you move from there.
So this is a.
an exciting very cool use case,
but it's not an imaging approach.
It's using this entire sensing system
to do measurements, but there's not
something that we currently target
because it's, it requires way more
engineering than making the imaging system
suitable for biology tests or something.
Dan: Yeah, my thoughts are, first
of all, with the semiconducting
world, you're dealing with precision
manufactured pieces of equipment.
You know exactly what to
expect where and when for every
single pixel as you put them.
With the biology use case,
That's way more dynamic, right?
My question is you'd still
be using the magnetic fields.
So what type of things would you be
looking for and able to detect, or
it may be that you're enhancing other
imaging techniques that they use maybe
to get another perspective on a, on what,
whatever's happening in the Petri dish.
Kevin: Yeah.
I think this is the big question
that is still at The, so I
would say academic level.
So there is no, and this is the big
difference to the semiconductor use case.
There is no current tool that is
in the field that clearly doesn't
perform at one of the challenges
that we could basically solve, right?
So that exactly, this is the question
that we also asked the biology labs
is what is the advantage of having
a non destructive measurement system
on the nanoscale that you would pay
for like half a million euros, right?
So there are very good approaches in
biology where you don't have nanoscale
imaging because they don't need nanoscale.
It's just like too good for them, right?
So they are not willing to pay for it.
I think this is something that we have
to see over time and describe what
additional insights can we generate?
And there it is not 100
percent clear what this is.
Dan: on the GNSS free or GPS free
type environment that's such a,
Exciting use case for lots of
different industries, shipping
underground metros defense and so on.
My understanding is that NV sensors
perhaps are not sensitive enough
for that one, but I think of
whichever one you use, you
mentioned SQUID, you mentioned, GMR.
So that's the classical.
Uh, magnetic field detection, isn't it?
And then OPMs as well.
And of course they some of them
are more sensitive, than NVs.
And I think that may mean that they're
more suitable to the navigation one.
But I'm not sure.
Kevin: Yeah.
So I think um, in general, this this
GPS denied environment navigation is
also what we are excited about to talk
about because it sounds pretty cool.
Yeah.
But the question is then
what is the use case?
Are we sensitive enough?
I know that the U.
S.
Department of Defense has looked into
this with a couple of tens of millions
of budget, and they couldn't get down to
10 or 20 meter off of precision, right?
So it's still like 200 meters
here and there you might be.
So it's really a kind of worst case
scenario where there's no GPS, and this
has to be the alternative to navigate.
So there is a lot of dual use and
some defense thinking that in the
worst case, this can be of help.
But as you said, in terms of
alternatives, I think NVs are not
inherently the ones you would basically
use for an alternative to GPS.
Dan: And that's, it's
an interesting topic.
I want to explore this
more, I think in due course.
With GNSS free environments, you, it's
not just about the magnetic field.
That's a very interesting data
set to work from, but it's also
your inertia and your movement.
And if you can somehow calculate that
very accurately, then you can cope with,
uh, just an occasional connection to the
GNSS or some kind of reference point.
Hey, I'm just spitballing
Kevin: No, but I totally agree.
And also there, I think the post
processing and this physics and for
machine learning can help to increase
the resolution or the sensitivity.
So this is something where on
the post processing, it can lead
to the fact that it actually
outperforms or is more attractive.
But so far we didn't look too deeply
into this just because It's a kind
of threat scenario that the entire
GPS system is not working anymore.
And then we would be
being the alternative.
I think it's a bit more pleasant to
think about a a civil use case where
there's a semiconductor manufacturing
that you can help to improve.
Steve: Yeah, and so one thing that
came to my mind now, you mentioned
using cheap GPU to help fix GPU.
But can you use quantum sensing to
enhance quantum computing, for example?
So these superconducting qubits need
to be manufactured in a way that keeps
the error rates of the qubits very low.
It's very important for, the
success of quantum computing.
Can we do quantum computing
in the first place?
So do you think your technology
could be used to help manufacture
quantum computer chips.
Kevin: Yeah.
So this is really off the
print, I would even say.
So I think there was a paper
published last week where they
used our quantum sensing approach
to actually manufacture chips.
So in the end no matter if you have
quantum computing chips or conventional
chips in the manufacturing process
it's a very similar approach.
We in general have been a bit cautious
to I would even say entangle quantum
sensing and quantum computing too much.
So I think it has pros and cons
from a funding perspective.
There's a lot of funding in quantum
and it, you get a lot of open doors.
If you say we do quantum because
there's a lot of interest in it.
But at the same time, we are so close
or like even in the industrial use cases
that people often connect quantum with in
10 years, something will happen, right?
So we're a bit careful there.
But of course there is in the
chip manufacturing, also the
manufacturing of chips for QC.
Steve: Yeah.
I see your point because, quantum
computing is not applicable right now.
So if you tie too close to the quantum
computing might be held back or in your
case, you have applications for today.
But uh, but I see that the same process,
the quantum chips, you would probably
even need more or fewer defects, let's
say than the classical technologies.
Because the error correction techniques
for quantum will be much harder to
overcome than, or to solve than the
classical ones that already exist.
Kevin: So we have that like in the
back, but right now, of course the
customer interest comes from more complex
architectures, also new materials.
We didn't talk about gallium
nitride, silicon carbide more
energy efficient material.
So I think before we.
focus on manufacturing
quantum computing chips.
There's still a lot of other
use cases within the semi
field that we would explore.
Dan: Yeah, I'd expect in the quantum
computing world, it's a totally
different ballgame, isn't it?
Because you've got to look at
Josephson junctions are probably the
elements that you'd have to measure.
And they have to be triggered somehow
with microwave, and they probably
need to be I don't even know whether
looking at the magnetic field is useful
in, in, in that manufacturing domain,
Kevin: Yeah.
Dan: it may
Kevin: Also there, it's very exciting, as
you said from a research perspective, but
there's no commercial use case right now.
So we monitor it.
We look at the papers, but
yeah, it's not top of mind.
That's it.
Dan: You want to go with a
high volume is as well, of
Kevin: Yes.
Steve: Okay.
So there's always an interest
about how companies get off the
ground and the paths that take
always shift and, making decisions.
So you had mentioned what the exist
program, which is a nice way to kick
off a company without having to risk
too much, because it's, you get The
stability from the university and the
salary, but have you quantum diamonds has
gone through the Intel Ignite program.
How do you think that has
helped the company kick off?
I guess it helps from our perspective.
It looks like it takes tech founders and
helps them to take their tech to business.
Is that something that helped
you or what's your opinion?
Kevin: Yeah.
So what I think about corporate
accelerator programs, first of
all, they don't take any equity.
So I think this is very important also
in terms of fundraising, that you're
not bound to any of of the corporates.
They have the requirement that
you need to have at least I
think 5 million in funding.
So they want to help you to Go
from five people to 20 or 25.
So this program had a lot of
components around team building,
onboarding how to manage as a CTO,
as a CEO, a deep tech company.
So from that perspective, I think it
was different than the, I would say,
usual programmers that we do a bit of
pitching and we reach out to investors.
It was really about.
You have the budget and you
have the office and the lab.
Like, how do you get to a
specific speed very quickly.
So they are there.
It helped definitely.
And the other part of the program is the
use case within Intel or the application
of the technology within Intel.
So they have a strong incentive.
to find the respective counterpart in the
organization and check on the technology.
So I cannot reveal that much,
but of course they also took our
technology and tried to see what is
the best use within the organization.
And this is something that we found
very helpful because if you think about
Intel as an organization, It's huge.
How do I get to that person?
Sometimes you don't even
understand their job titles.
So that really helped to understand where
can we be used and also where can we not
be used, where are some physical limits
or engineering issues, willingness to pay.
So all of this helped to come up
with a roadmap for the next two
years that is aligned with one
of the big potential customers.
Steve: I know one problem with startups
is especially coming from a university is
how to bring your product down to earth.
How do you make it a reality
soon as possible so that,
investors have interest in it.
Did it help shape your product?
You didn't mention it, but did you have,
let's say 90 percent of everything.
Ready to go.
And then they gave you some ideas
for 10 percent or did it really
help the company in a significant
way to pivot into the right market,
Kevin: in terms of funding or strategy or,
Steve: basic product development, product
roadmapping shaping the product so that
it could be sold in two years, five years.
Kevin: yeah.
So I think they had really had, because
in the end what I was trying to push
from the very beginning in particular
as a non quantum physicist is what
is the problem we're solving, right?
So we have these 15 quantum
physicists in the team.
They really like to read papers and
come up with new cool approaches.
And we, as a team have aligned that we
have the need to focus on the customer.
So there we, of course, talk to
them like what is the best solution?
And if you would have a wish list,
what would you actually need?
And align that with the product roadmap.
But I would also stress that it's
important to make sure that you don't
develop a product just for one customer.
So even if in the Intellignite program,
we got a lot of feedback where we
would have to be in terms of product.
It's there are still other
customers that you should talk to.
So I think it helped us, but we
were aware that there might be
the tendency to develop something
just for the one customer, just
because it was easy to get access.
Steve: Interesting.
Dan: Yeah, I love that.
You've got to think about risk
management and and so on a
very early stage, haven't you?
And not betting on one horse,
Kevin: Yeah, in particular and also this
is not not a surprise or something new.
It's a highly dense, consolidated industry
with a couple of big players, right?
So you can actually talk to
your top 20 customers in a year.
So it's not a consumer business.
Yeah.
So you have, if you go If you're
a supplier to, to let's say Intel
at some point, they bring you
in all of the different fabs.
So like talking to all of these 20
customers in the first place to, to align
your roadmap or something that's feasible
from a time and budget perspective.
Dan: Okay, well, let's, uh, let's wrap up.
Kevin, thank you very much for joining us.
That's been a fascinating talk
with you and little view into
the world of quantum sensing.
And I will watch your company with
with close interest in due course.
I wish you the best of luck.
Kevin: Thank you so much for having me.
Thank you.
Steve: Thanks a lot.
Dan: Bye for now.
I'd like to take this moment to thank
you for listening to the podcast.
Quantum networking is such a broad domain
especially considering the breadth of
quantum physics and quantum computing all
as an undercurrent easily to get sucked
into So much is still in the research
realm which can make it really tough for
a curious IT guy to know where to start.
So hit subscribe or follow me on your
podcast platform and I'll do my best
to bring you more prevalent topics
in the world of quantum networking.
Spread the word.
It would really help us out
Creators and Guests
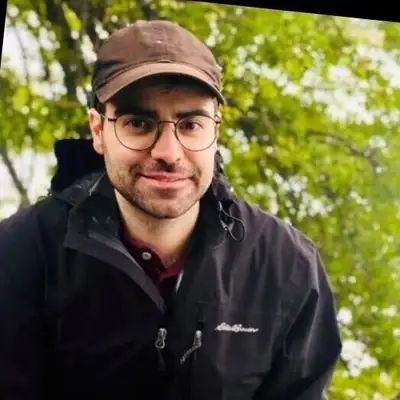
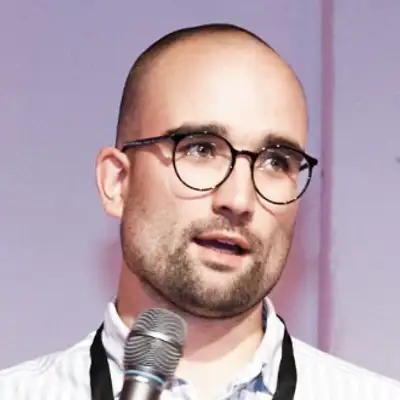
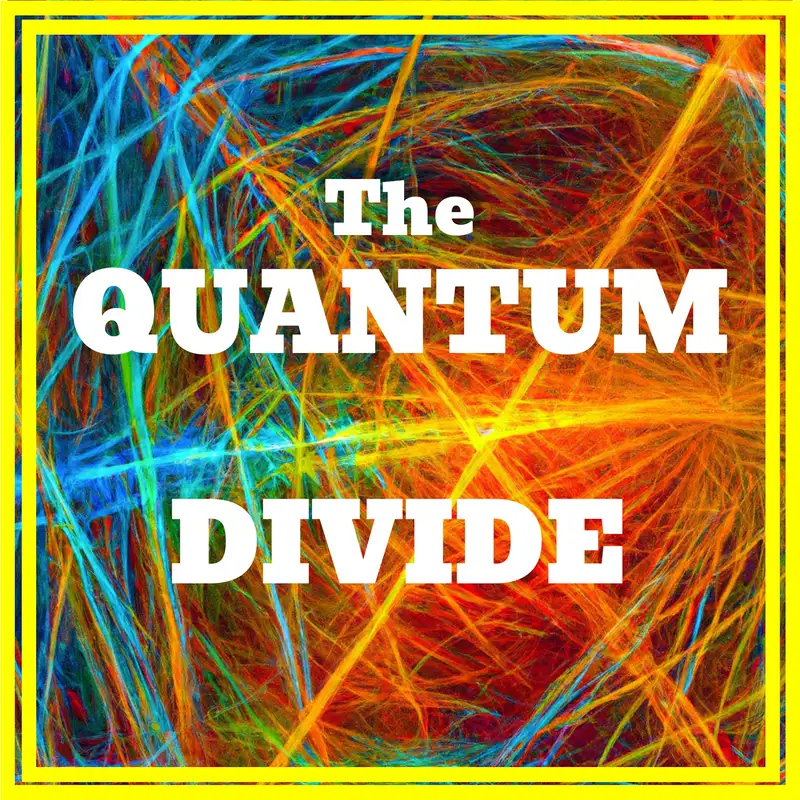