Distributed Quantum Computing
Dan: This is the quantum divide.
It's a podcast for people
curious about quantum networking.
What it means for the it industry
and what it might mean for them.
It's still early days for quantum,
but it's a very broad industry with
many different topics to understand.
The most imminent.
Our post quantum cryptography
and quantum key distribution.
Looking further ahead.
There are many academics building
ways to distribute content computers.
To leverage the power of
multiple machines over a network.
And how to manage quantum
state over that network.
Greetings I'm done home.
I'm a quantum curious technologist.
With no classical training
in quantum physics.
So basically that means.
I'll be the one asking
all the stupid questions.
For me, this podcast is a
vehicle to build my knowledge.
And I want to take you
on that journey with me.
Steve, second episode already I'm pretty
excited especially considering this topic.
So we've agreed we're gonna talk about
distributed quantum computing, and I know
you've done some work on this previously.
First of all, how you doing
and what you've been up to.
Steve: You haven't been doing well.
Finally somewhere in, in
Germany, so can go out for walks
and not be rained on or cold.
So that's a nice perk.
Dan: Always a winner.
Same in the uk.
Pretty much.
I think we got similar weather probably.
Steve: yeah.
Dan: Okay, mate.
Yeah you let me know about your a
project that you worked on previously
and a blog that you wrote about
distributed quantum computing.
I'll put the the link in the notes and the
show notes, people to read if they want.
But do you wanna give us an overview
of your view on this fascinating
topic and some of the research you
did and as usual, I'll question.
Steve: Yeah, sure.
So the idea is that when you're
building a quantum computer.
Let me phrase this properly.
You have to be careful
with these kind of topics.
When you're building a quantum
computer, the goal is to maximize
the number of qubits that can fit on
the quantum processing unit because
you have to contain these qubits
in this housing that protects it
from the environment in most cases.
This housing is not cheap and you
have to keep it very controlled.
And even things like outside
of the container influence
the quality of the cubits.
So things like vibrations in the
room, or vibrations in the ground
could be something like cars passing
by the building are enough to
trigger infidelities in the qubits.
So these things are very expensive
and they're very controlled.
And you have to keep them
in this small container.
And what that means is you're
limited to how many cubits
you can fit in that container.
Not only is you maximize the number of
qubits that you want in that container,
but it's a container and it's only so big.
Moreover the way you control
the cubits that are inside that
container need classical control
information coming from the outside.
So when you run a quantum algorithm, You
program the algorithm classically, so
that means it's converted from a code
to control logic, which then influences
the qubits using usually light pulses
that have to come in from the outside.
So those control signals
have a bandwidth as well.
You can't send unlimited amount
of control signals to one device.
So there's a lot of things that influence
the size of the quantum computer and what.
I think as a solution is that you can
take more than one of these containers
and QS put them side by side or put them
in the same room then put a interface
between them and network them together.
So now you have more than
one quantum computer, which
are more or less independent.
You put them side by side or
near each other so that you
can network them very easily.
And then you have to introduce, okay,
so now you have two quantum computers.
That could mean in theory twice the
power, which likely isn't the case.
It doesn't scale like that, but you can
think about having at least more qubits
and you solve the problem in a simpler
way than thinking about increasing
the size of the container or trying to
reduce the number of control signals,
or try to increase the bandwidth
that you have into the single device.
But you just take two copies
of what we already have.
And I'll work with those two
things independently, but then
network them together so we can
use them together at the same time.
Dan: Yeah.
And in the, in industry
at the moment, right?
There is from what I can tell there's
different levels of scaling being
implemented to improve quantum computing.
The first is obviously scaling up the
NISQ quantum computers, so improving
their fidelity, using error correction,
increasing the number of qubits and so on.
And what we're talking about
here is a bit like clustering.
In the traditional IT world it's
having multiple machines on a network
that can share a workload in this
case, some kind of algorithm or
what, whatever the workload is.
We're talking at quite a low level
at this time, somewhere down the
road where the computers are more
advanced, more stable this may be a
a good way to very quickly scale up.
If there's not necessarily a
threshold that's reached, but some
kind of technological limit in
scaling the quantum computer, of
which we are, from what I can tell,
breaching quite a few as time passes.
But ultimately in order to break
shore's algorithm it's like
feeling qubits or something.
And where we've got machines now.
I think IBM has some machines around.
Few hundred qubits.
So maybe even I think there are others
with different types of machines,
which are up to four, 5,000 qubits.
Correct me if I'm wrong there,
but I it's hard to compare them.
I know because they're all,
there's many different types of
implemented quantum computers, right?
Steve: That's, yeah, that's correct.
I think I, one thing I forgot to
mention also is these special containers
and the special environment that
these containers have to live in.
Because it's so hard to find the right
place for these quantum computers
to sit, then they tend to put them
more than one in the same housing.
So if you think of how IBM M has their
quantum computers, I think they have
the biggest one right now as something
like 477 or something like that.
I don't know if there's a thousand
Cuban machine yet, but I think
it's in their projections.
But anyway, so they tend to put
these computers in the same area,
physically locate, like physically
located close to each other.
But then the idea is, yeah, simply if we
were to network those quantum computers
together, the goal is to distribute
the workloads amongst those computers
because of the fact that there's not
enough qubits to execute the full
algorithm on a single quantum process.
Dan: Yeah, I think I got the
5,000 from D-Wave actually
they have a 2000 qubit chip.
I think and but it's a
different type of system, right?
There's quantum man kneeling as
opposed to trapped irons or so on.
So I, yeah, I guess back
to the whole apples and
Steve: Okay.
Yeah.
Yeah, that makes
Dan: but anyway, yeah.
I think it may be in a, would you
call the IBM machines, N machines
or how would you describe them?
Superconductor.
Steve: might even, they're super
conducting qubits, but I don't like, N
machine is tricky to say, like it's, of
course it is a N machine, but does it do
something yet that can be useful for us?
I would still say they're in this
prototyping stage where the quality of
the quantum computer is still before nsk.
My opinion.
So the question is does it do something
to improve what we can do currently?
I don't know, but it is,
in my opinion, not yet.
But so it's, it is technically nsk.
It's not air corrected, that's for sure.
Dan: Okay, let's I propose you do a,
an extra episode on on the different
types of quantum computers, but we've
gone on a tangent already, so Yeah.
Back to the distributed
quantum computing project.
Steve: Yeah.
Okay.
So the the problem with the connecting
quantum computers together, it's not
like you can just connect them together
and then you get everything for free.
You don't, like I said,
the scaling is not linear.
You just, you don't get just
two x or three x, the number of
quantum computers number together.
Is the factor of which power you have
more of, given that the quantum computers
are the same in the first place.
But Yeah, so the overhead that comes
with that is you need to communicate
between the quantum computers at a
minimum that's like the first stage.
So that alone costs time.
So communication is not free.
It comes at the speed of
light is not instantaneous.
And moreover, communication has
multiple layers and it's not like
you communicate and get everything
you need on the first message.
So there's lots of messaging
overhead involved with that.
So that's one part that's distributed
on computing that comes at a cost.
And then the second part is just
because you have a distributed quantum
computer doesn't mean you can run
the algorithms that you can run as
if there were one single computer.
There's a layer before that.
So when you write your algorithm in
KISS kit or something like that, or
some monolithic version, meaning , I
programmed my algorithm designing
it specifically for a quantum
computer that exists in one location.
Now taking that algorithm and taking it
to a distributed setting something again.
So there has to be an algorithm
that remaps that algorithm
to the distributed system.
And that algorithm can behave differently
depending on the topology of the
distributed system, the qubits in
the system for each device, how you
perform your classical communication.
If you need also an additional resource
of which I haven't mentioned yet, which
is entanglement between the devices.
And that's also another
communication cost is how much
entanglement you need to generate.
And that's also relies on which algorithm
you're using and how you network to
devices and what technologies you have.
My point is it definitely doesn't come
for free and it definitely doesn't give
you a linear factor of improvement, but
it gives you some improvement, let's say.
Dan: Yeah, for sure.
There's so many factors here.
We're talking about the different ways
of essentially sharing information
between the quantum computers, you
the options you've got, you mentioned
entanglement swapping but also
direct transmission of qubits that
we discussed in the previous report.
That's just one level of complication.
Then you've got the algorithm on top.
You've then got the what I think you
call the architecture of the circuits
that are need to be implemented.
So yeah.
I'd be interested to know about what
a control is cuz I see that comes up
in some of the resource requirement
trade-offs that that you talk about.
Steve: When we're talking
about control qubits.
There's, in each quantum processor,
there's what I classify as
two types of qubits at least.
There's the control qubit, and then
there's a communication qubits.
The communication qubit can be implemented
in different ways depending on how
the Quantum computers are built.
They're superconductor or they're made
with different technologies if the
qubits can move physically or not.
But in my picture is the cubits are
all static, and we have the control
qubit and the communication qubit.
And in order to do distributed quantum
computing, what you need to be able to
do a two cubic gate across two computers.
So if you're thinking about you have two
quantum computers sitting next to each
other and I need to perform a C knot gate
from one quantum computer to the next.
So how do you get the control information
from one computer to the other?
Can be done in a few ways, but the way
that seems to be most efficient to.
Personally establish entanglement
between the two quantum computers.
And to do that, we make use
of those communication qubits.
maybe a picture to think about is like
you have some partition of control qubits
and communication qubits, and then we
interact the control qubits with the
communication qubits on one side and do
the exact same thing on the other side.
So each quantum computer has communication
qubits and control qubits, and then
we Transmit the control information
from one computer to the next.
You have to perform this particular
operation, which involves generating
entanglement and classical communication.
So that's a bit complex.
Mean what to say in one paragraph.
Dan: Gonna say, where does the
classical communication come in?
Because
You talk about in the easiest way using a
control qubit, but is it not the easiest
way to just use the classical way as much
as possible because it's tried and tested.
Steve: I say the easiest because
technically I think both ways, like
both ways, I think of it as the
way that's called the cat entangle.
Cat disentangle.
This is slightly more efficient than
performing quantum teleportation.
Both involve classical
communication, both involve creating
entanglement between devices.
when you think of quantum teleportation,
it's a movement of the quantum
state , from one place to another.
So the classical communication
always has to be done.
But quantum teleportation involves
this movement of information
from one place to another.
And if you don't want to
accommodate that in your algorithm,
then you have to move it back.
So if you think, okay, this qubit, which
I label qubit A lives on machine A and
qubit B lives on machine B, and I wanna do
A C not gate between qubit A and qubit B.
And if you wanna use teleportation,
then you have to teleport cubit
A to machine B, perform your c
o gate between the two qubits.
And then you could have the option
of teleporting the qubit back or not.
And generally you want the qubit back
because it simplifies thinking about
the algorithm or else you have to keep
track of where that qubit is moved to so
that you can now make any modifications
to the algorithm in the future.
So if you put it back,
everything stays the same.
Everything is static again.
But if you move it it's on another place.
So with the cat entangle still use
classical communication and entanglement,
but the benefit is the qubit is
still in the same place as it was.
So the information the cubit is
holding, let's see is still static?
It's still on Cube, quantum,
Mach, cub still on machine.
A Yeah.
Dan: To me.
Steve: without pictures.
But
Dan: Yeah.
Yeah.
It's a good test, isn't it?
Steve: Yeah.
Dan: To me I would think that,
you'd want to dispose of that qubit.
Once you've done the c o gate, because
it's just complex to send it back again.
Come on, it's hard enough
to send it over once.
Surely.
Why do you need to restore it?
But I guess that I'm missing some
fundamental information there.
, Steve: but if you said destroy is the
things, you might need to use it again.
You might need that
information more than once.
That's the thing.
Dan: Next topic.
I just wanna, it's related for sure.
I'd like to just understand a bit
about cubic connectivity within a
machine and little bit about the
hardware and how the qubits are held.
And there's many ways of doing it, but
I've seen pictures of quantum CPUs, Qs
With X number of qubits on board, and I'm
imagining that there is essentially the
qubits are held in space somehow using
whatever field it is that is reacting with
the qubit and is able to hold it in space.
But then what kind of issues are there,
or perhaps what kind of Challenges are
there with getting qubits to interact
with each other inside a chip, because
if you're wanting to send qubits
between machines, that has quite a big
impact on and what you're gonna do.
And do you always hold those qubits in
the same place and use them forever?
Or do they have to be regenerated
every every now and again?
Steve: Yeah, so the connectivity
problem, firstly you said it
depends on the technology.
So superconducting chip is fixed.
They don't move around Physically,
what goes through them is some
currents and that current acts as
a qubit, but there's no movement.
That current is fixed , on the chip.
And when you have multiple qubits on
one chip, You need a way to, the goal
of quantum computing is interact, is to
make use of entanglement in some way.
So to get entanglement, you want
to use more than one qubit, and
therefore you need to make quantum
operations across more than one qubit.
So imagine you have a grid, let's
say it's three by three grid,
and you want to interact qubit at
the bottom left or the top right.
So in order to do that, generally what
you do, you shine some light at it
as microwave pulse, and if you shine
a microwave pulse across the whole
grid, you'll interact with all of
the cubits on the grid, potentially
causing errors on the other qubits.
So what you want to do is bring those
cubes close together somehow, and then
make a narrower beam so that you don't.
Interact with the remainder of the system,
and that's the connectivity problem is
bringing qubits close to each other.
Can you interact at all?
Qubit at the bottom
left with the top right.
In order to do that, you need to
make this procedure called a swap.
Not entanglement swap, but a swap.
It's a d it's a different concept.
It's just basically thinking about
alternating, position one with position
two, two goes to one, one goes to
two, and then you shuffle the cubits.
Around on the chip.
Not the cubit itself, not the
physical cubit, but the information
on the cubit gets shuffled
Dan: The state of it,
Steve: Exactly.
The state.
And that needs to be moved close to the
two interacting cubits so that light pulse
doesn't interact with the entire system.
It's more, it's a narrow beam.
And that's the connectivity is
can you actually bring those
cubits close to each other?
In order to perform two cubit operations.
And then I think the next part was
do you reset the system every time?
So there's a lifetime of the qubits and
the lifetime determines how big
of an algorithm you can run or how
long of an algorithm you can run.
So the lifetime of a qubit.
be something like this is hugely out of
orders of, let's say it's 10 seconds,
and if it takes one second to perform
a gate operation on the qubit, then
you can run 10 gates on the qubit.
And that's the extent of
your algorithm, let's say.
So then after, 10 operations, your
qubit state becomes a mixed date,
and it's just a random output.
It has no meaning.
And that's that's the problem.
So the goal is to extend the
lifetime of the qubits so you
can put more operations in one go
yeah.
So that's one goal to reach.
And also is to shrink the duration
of the gates so you can have kind
of two parameters to work with.
If you can run the gate in half a second
instead of one second, then you also
increase the size of the algorithm.
But after the extent of the lifetime, like
when the lifetime of the cubit expires,
then of course you have to reset and redo.
That's quantum anyways.
You always have to repeat the process many
times to get the statistics of the system.
Reset the system, run the algorithm,
reset the system, run the algorithm.
It's, yeah, that's usually how it goes.
Dan: So the magic of the hardware is being
able to, I think of it as spinning up
the state that's necessary and performing
all of the tasks that are necessary in
advance of implementing the circuit.
And the bigger the grid of qubits,
the more complicated that is.
Steve: Yeah, that's correct.
Exactly.
So if you have bad connectivity, let's
say, then you have to add a lot more
additional gates to your algorithm only
for moving the qubit information around.
That actually that's not about the
algorithm, that's just about bringing
the cubic close to each other so
that you can perform to cubic gates.
So it's adding additional logic that
doesn't perform anything meaningful
and you lose in that sense too.
So the larger your algorithm, larger
the circuits, that means the larger
the quantum processor, the more gates
you need just for moving things around.
But also, like you said, one
thing is spinning up the state.
That's one part of the algorithm.
That I didn't mention
actually, state preparation.
So bringing the qubits into the
state you need in order to operate
on them in the first place also
contributes to the lifetime of,
the duration of the algorithm.
And generally what is in a
lot of cases, especially when
it comes to loading classical
information onto the quantum state.
by the time you load the classical
information, the lifetime of the cubit is
already finished and you don't even get a
chance to perform your algorithm because
loading information also is costly.
So that's, there's a lot of problems.
Cubic lifetime, the longer it is, the
better, but there's so many things
involved that occupy time that isn't
even related to the algorithm itself.
Dan: Yeah, of course.
And then now let's zoom out a
little bit again and go back to
the distributed computing example.
You spoke about remapping,
monolithic circuits.
And I think of that as a bit like when
you take a monolithic application and
you refactor it to your microservices
architecture and you're essentially
putting different services on
different machines different locations.
It's clear that there's a whole bunch of
other things you have to build into it.
The entanglement between ppu, perhaps
what other things do you have to take
into account when you're developing
the way a circuit is gonna be
distributed across multiple machines.
Steve: Yeah, so as a user, ultimate
goal would be that they wouldn't have
to consider those things themselves.
But when you are writing the compilers
and things like that, then of course
every then it has to be considered.
That's for sure.
And some of the things I think
are important of course, is
conductivity of the architecture.
So you don't wanna continuously use
qubits that are far away from each
other, that always have two cubic
K next to each other, for example.
If you have a quantum algorithm that
has a lot of two cubic gates between.
Two cubits that are, then when you make
that remapping, you should try as best
as possible to keep those two cubits
on the same device in the first place.
will reduce the classical
communication overhead.
And then again, what you think when
you go to the layer of the single
quantum computer is make sure that
those cubits are close to each
other physically on the chip itself.
So localize 'em to the same
quantum computer and localize
them on the quantum chip.
So that's one part.
And that's what a compiler
should think about when it's
executing that compilation.
Dan: Going across the network, right?
I guess there's ultimately some kind of
atom light interaction where you need
to take either entanglement between
a qubit on a chip to something that's
being sent across the, flying qubit.
And then the same process has to
happen in reverse on the other end.
Steve: This is like
transduction as far as I know.
It's taking the quantum state,
bringing it to a flag cubit.
So there's two, there's
a couple things there.
So it's the entanglement.
So you could do something
like creating entanglement.
So there's still, there's needs to be
transduction done, but you can do it with.
Communication, bits like that
don't contain information.
So you can establish entanglement without
having to think about interfering with
the information containing qubits.
But you still need transactors, so you
need to create a flank cubit so that
you can generate entanglement between
devices at that's also very costly.
It's not deterministic.
You don't always get that on
your first attempt, and that's
another thing to consider is.
As you're establishing entanglement,
those cubit lifetimes are deteriorating
right time, as time goes on.
So everything has to be done fast and
everything has to be done without
failing as much as possible.
I lost my train of thought though.
I think we were talking
about what goes into.
Dan: It's very easy to do.
I am listening to you talk.
I'm constantly losing my train of thought.
The layers of complexity we're
describing are they're quite
mind-bending, really in, I know we,
we have all these different layers
of the way our computers and networks
use in the real world at the moment.
And ultimately with looking at different
ways of creating the same kind of stack,
with , in chip Distributed behaviors
and everything that goes with that.
And then the whole control
layer over the top of that.
so yeah, it is
Steve: a lot to it.
Yeah.
Now that I think about it, yeah.
Dan: That's the fun of talking
about it, I think in this way.
So what about the let's
go to the control side.
If you're gonna, let's say you're in
a world where we have the technology
to take a monolithic circuit, spread
it across three Qs, you need some kind
of scheduler Or a controller that is
going to decide what that looks like.
And I think of that as
a bit like a scheduler.
You get in an operating system that
schedule different tasks down to the cpu.
And then of course you've got
scheduling or thread management
at the actual CPU itself.
So would you have those two kind of same
processes in is that a good analogy?
Steve: I think so, I think this problem
orchestrating the quantum computers
is completely classical problem.
It's just resource management in a sense.
There's nothing quantum
about the operating system.
It's just.
Making sure the resources are there
when they're needed and making sure
there's no overlapping of resource,
like requirement or something.
Not, no two quantum algorithms are using
the same resource at the same time.
And that's same thing in classical.
I think it's cause they give
it like files or something.
Not writing to the same file,
not modifying the same file.
Make sure that there's, thinking about
the ram and the quantum computer, that
, no two programs are using the same memory.
So I think it's exactly
analogous to that, like you said.
Dan: Yes, it's gonna take
the runtime schedule and.
Ensure that each PPU is ready
for it as freed up the resources.
I could also draw an analogy
to time sensitive networking.
We have control
Steve: Oh yeah.
Yeah.
Dan: In the center of the network,
which the switches devices route.
Reserve make sure that traffic is
prioritized, or in this case, maybe
ensure that the keep user ready to
send and receive at the right time and
everything can happen with that hitch.
Okay.
Steve: One thing I can talk a bit
more, also, one thing I like about
distributed quantum computing.
I have parallel quantum computing
and distributed quantum computing and
parallel quantum computing to me means
that, Two quantum computers are working
together to solve one problem, but
they don't interact with each other.
So it's basically parallelization of
the quantum algorithm with no interaction
until the classical outputs come.
And that's actually another thing that
you can gain by networking quantum
computers together, let's say networking
in quotes, because they're not actually
talking to each other, , you only
merge the outputs in a clever way.
My point is, you can run a quantum
algorithm on multiple devices
without the devices interacting
with each other, and that's what
I call parallel quantum computing.
And that's a much, much simpler thing.
That's nothing that we can't do today.
We couldn't already run that.
And probably they already do.
So that's, but that's nothing
but classical communication.
Dan: So in that example, I
think it's just to recap your.
Ultimately running multiple quantum
algorithms on some data or solving some
particular problem with an algorithm.
But you need to perform this multiple
times on different data sets or something.
And you're merging the information
then classically in whatever
application you've got in the end.
Yeah, that's just scaling, isn't it?
That's just using more resources.
But from what I can tell, you know the
speed at which these algorithms was done.
If you had one quantum computer,
then just hit one, and then another
one and another one you're probably
still gonna be able to serve that need
within a very short period of time.
Do you know of any use cases
where you might need parallel
computing in that way?
Where
you need to perform The
algorithms at the same time?
Steve: Yeah there's this one in
the paper we wrote some years back,
a couple years ago, listed three
different ones that were well suited.
But I think of the three that we listed,
the best one was the Variational Quantum.
I can solver that one is quite well used.
I think it's.
One of the driving algorithms of quantum
computing or quantum simulation at least.
And so in various quantum, I can solver,
usually you have this system, Hamiltonian.
It's operation that dictates
how the system moves with time.
And that Hamiltonian can
be rewritten in a way.
That it's a sum of operations.
So you take one operation and you
break it into a sum of operations.
And because it's like a linear sum,
you can run each of the parts of the
sum one at a time, or you can run
them all at the same time in parallel.
And then you, after you run this
operation over each part of the
sum, You have to merge the sum back
together, and you have one output.
So you take something singular,
break it into many pieces, execute
the many pieces, and then merge them
back together for a single output.
And how you break them up into
pieces how you execute the pieces.
Can do it in series, you
can do it in parallel.
That's not important, but it's, it
could be done in parallel and that would
basically speed up the run time, not
the complexity of the algorithm, but.
This time in which basically
increased number of resources
in order to reduce the run time.
Yeah
Dan: Yeah.
So what was the use case
though, that you mentioned?
The solar.
What's that?
Steve: It's generally used in chemistry,
finding the energy level, of molecules
. The usual use case is finding the ground
state energy of a particular molecule
and a molecule has a Hamiltonian,
and then you plug this Hamiltonian
formula into the VQ E and VQ E is then
used to minimize the energy level.
You get an estimate of the
ground state energy, which is
important for like drug discovery.
I'm not so familiar with that
stuff, but as much as I know.
Dan: Don't Worry, you've lost me already,
but no, I, I know that it's a big pharma
and, this is one of the big areas where
it's seen that quantum computing is going
to provide biggest impact first of all
in simulating chemical systems, right?
And interaction behavior.
I think we'll probably do another
pod on this one, but I want
to ask you about simulation.
Simulation of distributed
quantum computing.
My understanding that simulation
is, simulating the quantum
interactions, the quantum computing
interactions and the execution of the.
Circuits in a classical computer.
Is that right?
And is it the same concept when you
want to build a prototype simulation
of a distributed quantum computer
using some distributed quantum network?
Steve: Yeah, I'd say the
concept just extends.
So when you stimulate a quantum
computer on a classical machine
it's about tracking the quantum
state using the representations
that we have for qubits and try to
do that as efficiently as possible.
And then there's a matrix multiplication.
And that's what you do to simulate a
quantum computer on a classical machine
if you wanna do distributed quantum
computing simulation, then on top of that
what comes is the communication layer.
And the communication layer is the
way we simulate it is we use either
multi-process programs or multi-threaded
programs to mimic one device per
thread or one device per process.
And then the communication
between processes is what
needs to be added in reality.
So you can simulate the communication
layer by basically parallelizing the
quantum computing simulations and then
add the communication layer using.
Something to enable the discussion
of messages between processes.
Dan: So I'm thinking the, you're
not really simulating the quantum
Behavior that's required
for quantum networking.
There.
You're just sharing information
between the processes, which
are simulating the quantum,
Steve: That's true.
Yeah.
That's for noiseless simulation.
I guess that's depends on what
you're interested in discovering.
But that's one thing to do is
if you just wanna know, does
my algorithm work in principle?
If I had perfect quantum computers,
does this at least solve the
problem I'm trying to solve?
But there are definitely
ways to go one layer lower.
You can look at the noise
effects using simulation too.
Yeah that's could be done using
different techniques I think.
Yeah.
Probably, I think it has been done too.
I think I can think of at least one paper.
Dan: Okay.
That's interesting.
Yeah.
We'll put that in the show notes.
Yeah.
Another analogy from me.
I see simulating the quantum computers,
In a distributed sense a bit like the
way digital twins work these days.
The digital twins are, simulations of a
particular environment, many different
types in different industry verticals, but
a lot of them will have applications that
are talking to each other and they can get
very complex, but, One thing they tend not
to do is actually simulate the networks
that connects all of the devices, right?
It's just to give them that each
of the applications that performing
the process, whatever that is can
connect with each other and so on.
So yeah, that's just another
kind of strange analogy from
me, but that's how I see it.
Steve: Yeah.
There's so many questions to ask about
distributed quantum computing you can
implement the simulation in many ways,
I think, and it all comes down to what's
the question you're trying to answer?
I mean for me personally, , I'm always
curious about does the algorithm
actually distribute and how much
communication is involved when
executing on a particular topology.
So that's, so that is
above the physical error.
But probably a physicist would
be interested in thinking about
what noise tolerance do I have?
How long can the algorithm
run and what's the physical
properties of the system like?
That's maybe, it depends on the person.
It depends on the question, and
there's so many ways to do it though.
Dan: So you said this is a
niche part of the industry.
Because a lot of effort is going into
scaling and improving quantum computers
as a priority, which is understandable.
What do you see happening with
distributed quantum computing
looking forward maybe 2, 3, 5 years.
Steve: I think within two
years, probably nothing.
I would say in five years maybe
there would be the first experiment.
Lemme correct myself.
There was a experiment done, I
think two years or three years ago.
Within within three years Of
executing a distributed c o
gate over 50 meters of fiber.
So that's the first step of
distributed quantum computing.
So in theory, someone has done a
distributed quantum algorithm, at
least if you call one C o gate an
algorithm, which is still very impressive
and it's still very challenging.
So two static qubits connected with
a fiber, and then they put a flying
qubit, which interacts with the static
qubit into the fiber to bring the
control information across devices.
That actually is no entanglement.
And the way they did that was
quite interesting cuz I didn't
know about that approach.
It could simplify a lot because if you
don't need to generate entanglement,
then you save a lot of resources.
Dan: But then you have to rely on
the lossiness of direct transmission,
Steve: Yeah
Dan: the difficulty that comes with that.
Steve: Yes.
Yeah, that's the trade off.
But so anyways, to get back to
the question about the timelines,
I think in within five years,
maybe a more complicated system,
something like connecting to IBM
quantum computers will be seen.
I suspect that will be done in five years.
But I think it's still the
main goal is to make a quantum
computer that at least works.
Individually, and that's why
most people just focus on that.
So there's still so many open problems
with monolithic quantum computing that
distributed quantum computing is on
the back burner right now, but it'll,
I think it has to come eventually.
I believe it's inevitable maybe in
10, 15, 20 years, if there's one good
quantum computer, then the next step
is to just network them together and
it'll come with certainty if we can do
quantum computing on a monolithic level.
Dan: Makes sense for it to come.
When you think about economies of scale
and maybe efficiency diminishing, the
larger the computing system gets, the
individual computing system, then it
seems even if it's not something that
lasts permanently It, often there's like
an intermediary period where that might
be the most cost effective thing to do.
Cost not always coming into it, but
I know it's an important factor,
especially with commercial applications.
Okay, Steve, listen, that was
totally fascinating and I have to
go lie down in the dark room now
for a few minutes just to recover.
loads of questions as usual.
I am sure we've got much, much
more to talk about on these topics.
Thanks very much.
Steve: Yep.
Thank you.
And great chatting.
Dan: I'd like to take this moment to
thank you for listening to the podcast.
Quantum networking is such a broad domain
especially considering the breadth of
quantum physics and quantum computing all
as an undercurrent easily to get sucked
into So much is still in the research
realm which can make it really tough for
a curious it guy to know where to start.
So hit subscribe or follow me on your
podcast platform and I'll do my best
to bring you more prevalent topics
in the world of quantum networking.
Spread the word.
It would really help us out.
Creators and Guests
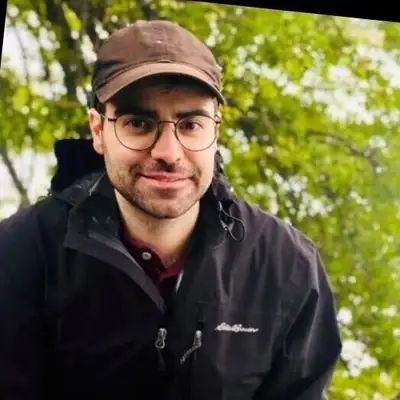
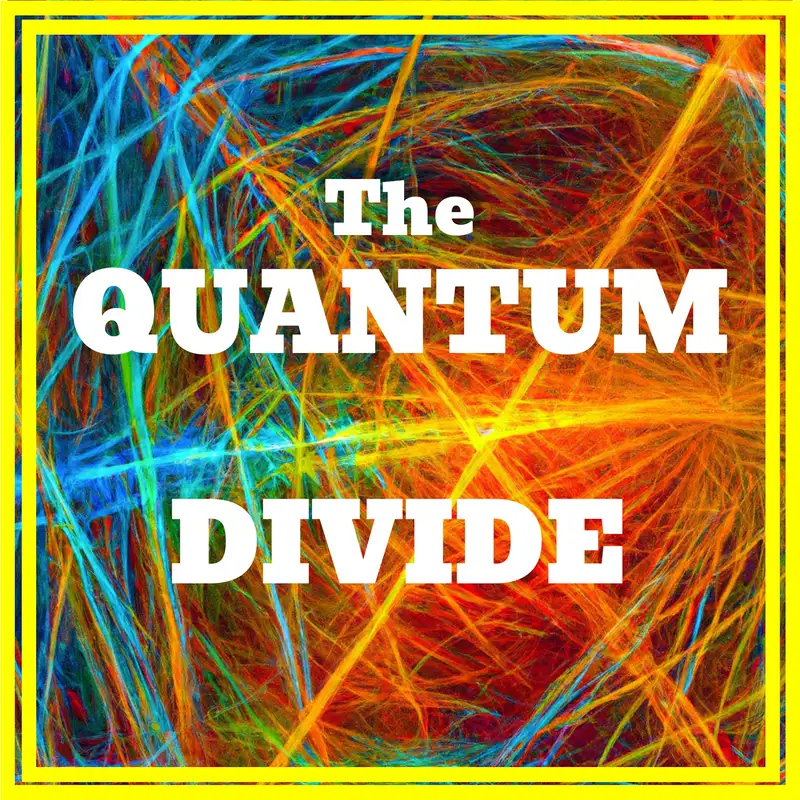